Random forest classification system based on kernel extreme learning machine and parallelization
A technology of nuclear extreme learning machine and random forest classification, which is applied in the field of random forest classification system and can solve infeasible problems
- Summary
- Abstract
- Description
- Claims
- Application Information
AI Technical Summary
Problems solved by technology
Method used
Image
Examples
Embodiment Construction
[0024] The invention adopts the extreme learning machine with mixed core as the base classifier of the random forest and optimizes the base classifier by means of sorting and particle swarm optimization, hoping to achieve better classification performance. System architecture such as figure 1 As shown, it contains the stand-alone module and the parallelization module.
[0025] 1. Stand-alone module
[0026] 1.1 Data extraction module
[0027] The sample set is sampled by the Bootstrap method, and N samples are randomly selected from the N samples with replacement to form a new sample set. The samples are coronary heart disease data samples, and the unselected samples form a test set. In the new sample set Randomly select f features (fi and the test set T i (i=1,2...k, k is the number of base classifiers). At the same time, the data extraction interface Bootstrap is set in this module, and user-defined data extraction methods can be used according to different needs to make...
PUM
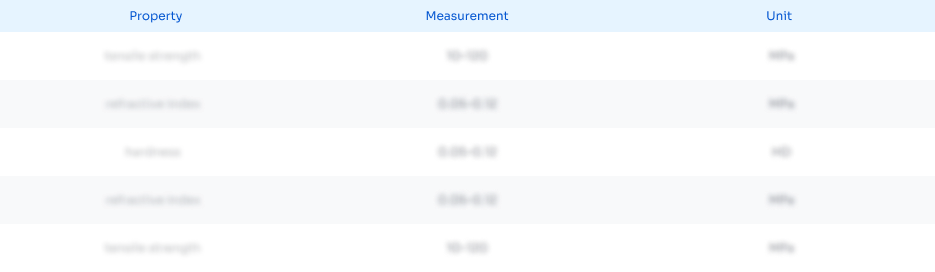
Abstract
Description
Claims
Application Information
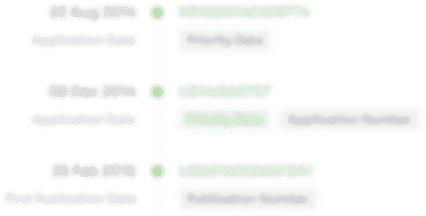
- R&D Engineer
- R&D Manager
- IP Professional
- Industry Leading Data Capabilities
- Powerful AI technology
- Patent DNA Extraction
Browse by: Latest US Patents, China's latest patents, Technical Efficacy Thesaurus, Application Domain, Technology Topic.
© 2024 PatSnap. All rights reserved.Legal|Privacy policy|Modern Slavery Act Transparency Statement|Sitemap