Method for generating adversarial image
An image and original image technology, applied in the field of generating confrontation images, can solve the problems of high accuracy of deep neural networks, and achieve the effect of reducing coupling, improving accuracy and good robustness
- Summary
- Abstract
- Description
- Claims
- Application Information
AI Technical Summary
Problems solved by technology
Method used
Image
Examples
example 1
[0170] Example 1, based on the Inc-v3, Inc-v4, IncRes-v2 and Res-152 models, generate L ∞ Constrained Adversarial Images for Untargeted Attacks.
[0171] The method provided by the present invention may be called a momentum-based iterative fast gradient sign method (MomentumIteration Fast Gradient Sign Method, MI-FGSM for short). In the process of generating images used in confrontation training, the noise threshold ε=16, the number of iterations T is 10, and the attenuation coefficient of the momentum item μ=1.0.
[0172] The Fast Gradient Sign Method (FGSM for short) without momentum term and the Iteration Fast Gradient Sign Method (I-FGSM for short) are compared with the method provided by the present invention.
[0173] MI-FGSM, FGSM and I-FGSM can also be called attack methods of deep neural networks.
[0174] Using models based on Inc-v3, Inc-v4, IncRes-v2 and Res-152 to generate L ∞ Constrained images used in adversarial training for untargeted attacks, attacking Inc...
example 2
[0178] Example 2, based on the Inc-v3, Inc-v4, IncRes-v2 and Res-152 models, generate L 2 Constrained Adversarial Images for Untargeted Attacks.
[0179] The method provided by the present invention may be called a momentum-based iterative fast gradient method (Momentum Iteration Fast Gradient Method, MI-FGM for short). In the process of generating an adversarial image, the noise threshold n is the dimension of the original image, the number of iterations T is 10, and the attenuation coefficient of the momentum item μ=1.0.
[0180] The Fast Gradient Method (FGM for short) without momentum term and the Iteration Fast Gradient Method (I-FGM for short) are compared with the method provided by the present invention.
[0181] MI-FGM, FGM, and I-FGM can also be called attack methods of deep neural networks.
[0182] Using models based on Inc-v3, Inc-v4, IncRes-v2 and Res-152 to generate L 2 Constrained images used in adversarial training for untargeted attacks, attacking Inc-v3...
example 3
[0186] Example 3: Integrate any three of the Inc-v3, Inc-v4, IncRes-v2 and Res-152 models with a neural network to obtain an integrated model. The weight of each model is equal, and the unintegrated model is used as the corresponding integrated model black box model. Obtain the loss of the integrated model according to the unnormalized probability, predicted probability and loss respectively, and generate the loss satisfying L ∞ Constrained Adversarial Images for Untargeted Attacks. In the process of generating adversarial images, the noise threshold ε=16, the number of iterations T is 20, and the attenuation coefficient of the momentum item μ=1.0.
[0187] The Inc-v3, Inc-v4, IncRes-v2 and Res-152 models are respectively used as black box models, and the corresponding training samples are used to attack the integrated model and the black box model, and the attack success rate obtained is shown in Table 3.
[0188] Table 3 satisfies L ∞ Constrained Attack Success Rate of Ad...
PUM
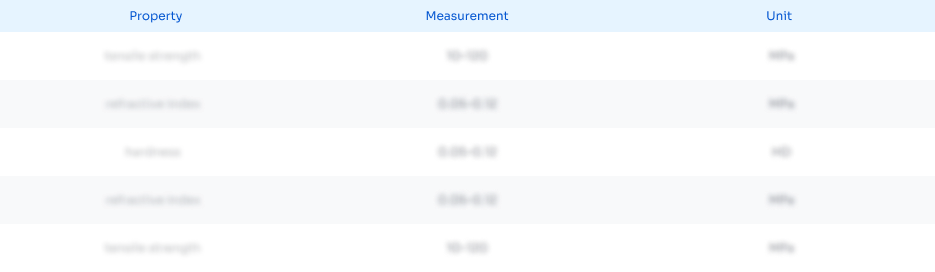
Abstract
Description
Claims
Application Information
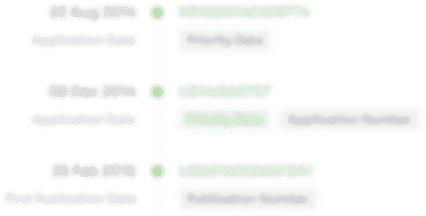
- R&D Engineer
- R&D Manager
- IP Professional
- Industry Leading Data Capabilities
- Powerful AI technology
- Patent DNA Extraction
Browse by: Latest US Patents, China's latest patents, Technical Efficacy Thesaurus, Application Domain, Technology Topic, Popular Technical Reports.
© 2024 PatSnap. All rights reserved.Legal|Privacy policy|Modern Slavery Act Transparency Statement|Sitemap|About US| Contact US: help@patsnap.com