Asynchronous multi-sensor space-time deviation joint estimation and compensation method and device
A multi-sensor, joint estimation technology, applied in complex mathematical operations and other directions, can solve the problem of effective estimation method and device for asynchronous sensor space-time deviation, etc., and achieve the effect of effective estimation and compensation
- Summary
- Abstract
- Description
- Claims
- Application Information
AI Technical Summary
Problems solved by technology
Method used
Image
Examples
Embodiment 1
[0066] Such as figure 1 As shown, wherein the joint estimation and compensation method of the space-time deviation of the asynchronous sensor includes:
[0067] Step a, use the filtering algorithm to estimate the target state, determine the estimated state and the estimated state error covariance matrix at k-1 fusion time, and calculate the state sampling points and corresponding weights at k-1 fusion time;
[0068] In this step, the UKF filter algorithm is used to estimate the target state, which has high precision and good stability.
[0069] UKF (Unscented Kalman Filter), the Chinese interpretation is lossless Kalman filter, unscented Kalman filter or dearomatic Kalman filter. It is a combination of lossless transformation (UT) and standard Kalman filtering system, through lossless transformation, the nonlinear system equation is suitable for the standard Kalman filtering system under the linear assumption.
[0070] Among them, the insensitive transformation is used to ca...
Embodiment 2
[0080] As described above, the asynchronous multi-sensor space-time deviation joint estimation and compensation method, the difference of this embodiment is that in the step a, the specific calculation formula of the state sampling point at k-1 fusion time and the corresponding weight value for:
[0081]
[0082] Among them, the number of state sampling points is 2m in total,
[0083] In the formula, ξ is the state sampling point, G is the weight; ξ j (k-1|k-1) and G j They are the jth state sampling point and weight at the k-1th fusion moment, k represents the serial number of the current fusion moment, m is the dimension of the state vector, and λ is the estimated value used to determine the k-1 fusion moment The scale parameter of the distribution state of the surrounding state sampling point ξ, and satisfy (m+λ)≠0; for The jth row or jth column of ; and P(k-1|k-1) are the estimated state and estimated state error of the expanded dimension state vector A(k-1) an...
Embodiment 3
[0096] The difference between this embodiment and the asynchronous multi-sensor space-time bias joint estimation and compensation method described above is that in the step b, for the predicted state sampling point ξ j (k|k-1), predicted state and predicted state error covariance matrix P(k|k-1); where the predicted state sampling point ξ j (k|k-1) represents the state sampling point ξ based on the k-1 fusion moment j(k-1|k-1) Prediction of the state sampling point at the kth fusion moment, predicting the state Represents the state estimation based on the k-1th fusion moment For the prediction of the state at the kth fusion moment, the predicted state error covariance matrix P(k|k-1) means that the covariance matrix estimation P(k-1|k-1) based on the k-1th fusion moment Prediction of the state error covariance matrix at k fusion moments; the left side of the vertical line represents that the prediction process is based on the conditions at the k-1th fusion moment, and th...
PUM
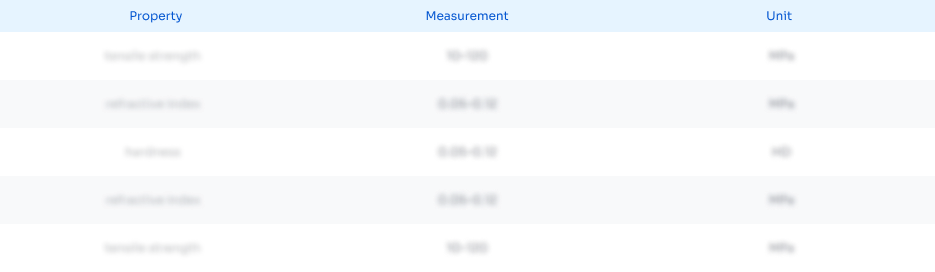
Abstract
Description
Claims
Application Information
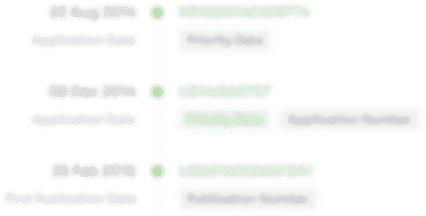
- Generate Ideas
- Intellectual Property
- Life Sciences
- Materials
- Tech Scout
- Unparalleled Data Quality
- Higher Quality Content
- 60% Fewer Hallucinations
Browse by: Latest US Patents, China's latest patents, Technical Efficacy Thesaurus, Application Domain, Technology Topic, Popular Technical Reports.
© 2025 PatSnap. All rights reserved.Legal|Privacy policy|Modern Slavery Act Transparency Statement|Sitemap|About US| Contact US: help@patsnap.com