Object Detection Method of Optical Remote Sensing Image Based on Multi-scale Feature Learning
An optical remote sensing image and multi-scale feature technology, applied in the field of image processing, can solve problems such as uneven distribution of target features at different scales, low detection accuracy of small targets, and inability to train parameters as a whole, and achieve rich shallow detail feature information, The effect of overcoming the imbalance of feature distribution and improving the accuracy rate
- Summary
- Abstract
- Description
- Claims
- Application Information
AI Technical Summary
Problems solved by technology
Method used
Image
Examples
Embodiment Construction
[0057] The present invention will be described in further detail below in conjunction with the accompanying drawings.
[0058] Refer to attached figure 1 , the steps of the present invention are further described in detail.
[0059] Step 1, construct a multi-scale feature network.
[0060] Construct a 31-layer multi-scale feature network, and its structure is as follows: input layer → first convolutional layer → second convolutional layer → first pooling layer → third convolutional layer → fourth volume Product layer → second pooling layer → fifth convolutional layer → sixth convolutional layer → seventh convolutional layer → third pooling layer → eighth convolutional layer → ninth convolutional layer Layer → Tenth Convolutional Layer → First Connection Layer → Fourth Pooling Layer → Eleventh Convolutional Layer → Twelfth Convolutional Layer → Thirteenth Convolutional Layer → Fifth Pooling Layer → Fourteenth Convolutional Layer → Fifteenth Convolutional Layer → Sixteenth Co...
PUM
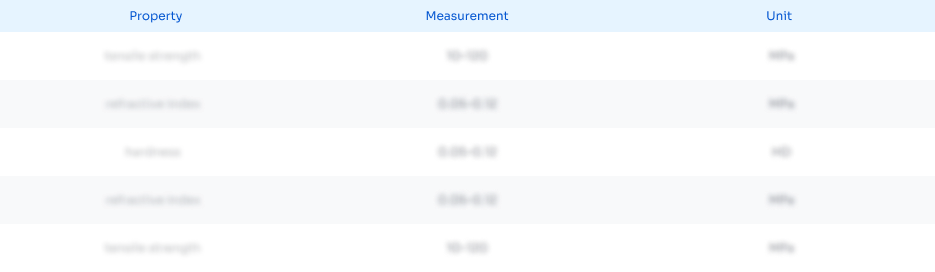
Abstract
Description
Claims
Application Information
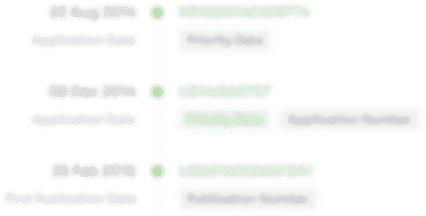
- R&D Engineer
- R&D Manager
- IP Professional
- Industry Leading Data Capabilities
- Powerful AI technology
- Patent DNA Extraction
Browse by: Latest US Patents, China's latest patents, Technical Efficacy Thesaurus, Application Domain, Technology Topic, Popular Technical Reports.
© 2024 PatSnap. All rights reserved.Legal|Privacy policy|Modern Slavery Act Transparency Statement|Sitemap|About US| Contact US: help@patsnap.com