Magnetic resonance multi-channel reconstruction method based on deep learning and data self-consistence
A technology of deep learning and magnetic resonance, applied in magnetic resonance measurement, measurement using nuclear magnetic resonance image system, measurement of magnetic variables, etc., can solve the problems of low acceleration factor, low processing capacity of high-magnification undersampling data, etc., to improve quality , improve the generalization ability and shorten the time
- Summary
- Abstract
- Description
- Claims
- Application Information
AI Technical Summary
Problems solved by technology
Method used
Image
Examples
Embodiment 1
[0044] A method for magnetic resonance multi-channel reconstruction based on deep learning and data self-consistency, comprising the following steps:
[0045] Step 1: Collect the K-space data of the multi-channel fully-sampled training set, convert the under-sampled K-space data into convoluted images as the input of the reconstruction network, and use the fully-sampled K-space data as the training marked data of the reconstruction network and based on Fully sampled K-space data to generate a convolution kernel;
[0046] Step 2: After inputting the convoluted image and fusing the undersampled K-space data and the convolution kernel, the reconstruction network constructed by repeatedly superimposing the SC layer, CNN network, and DC layer takes the training marked data as the target, and trains the network through backpropagation The parameters are obtained to reconstruct the mapping relationship between the input and output of the network;
[0047] Step 3: Input the undersamp...
Embodiment 2
[0050] Step 1 includes the following steps:
[0051] Step 1.1: Use multi-channel receiving coils to collect fully sampled training set K-space data, and generate under-sampled K-space data S through artificial under-sampling u , whose size is N x *N y *N c , where N x Represents the number of rows of collected data, N y Represents the number of columns of data, N c Represents the number of receiving channels; artificial undersampling does not require manual operation, and can be automated with a variety of algorithms; in the training phase, the undersampling data is generated by discarding some of the full sampling data; in the testing phase, the magnetic resonance scanner Undersampled data can be obtained directly; a full sampled data can only have one corresponding undersampled data, so after obtaining a full sampled data in actual operation, artificially undersampled to generate undersampled data on this basis.
[0052] Step 1.2: Undersampling K-space data S u Convol...
PUM
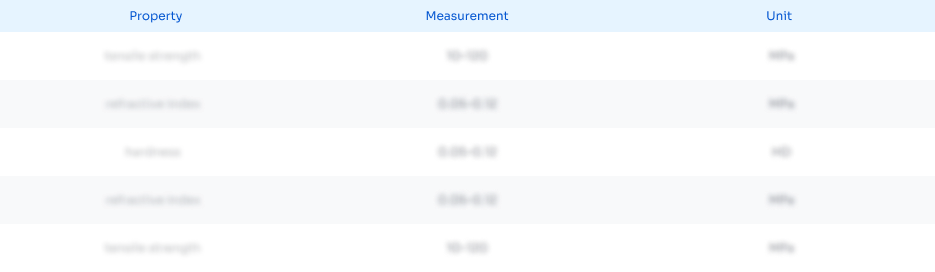
Abstract
Description
Claims
Application Information
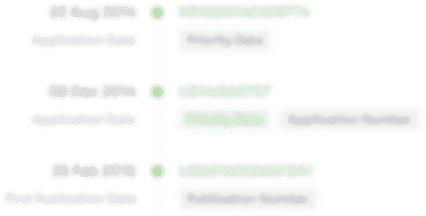
- R&D Engineer
- R&D Manager
- IP Professional
- Industry Leading Data Capabilities
- Powerful AI technology
- Patent DNA Extraction
Browse by: Latest US Patents, China's latest patents, Technical Efficacy Thesaurus, Application Domain, Technology Topic, Popular Technical Reports.
© 2024 PatSnap. All rights reserved.Legal|Privacy policy|Modern Slavery Act Transparency Statement|Sitemap|About US| Contact US: help@patsnap.com