A MRI multi-channel reconstruction method based on deep learning and self-consistent data
A technology of deep learning and magnetic resonance, applied in magnetic resonance measurement, measurement using nuclear magnetic resonance image system, measurement of magnetic variables, etc., can solve the problems of low acceleration factor, low processing capacity of high-magnification undersampling data, etc., to improve quality , improve the generalization ability, and the effect of accurate end-to-end mapping relationship
- Summary
- Abstract
- Description
- Claims
- Application Information
AI Technical Summary
Problems solved by technology
Method used
Image
Examples
Embodiment 1
[0044] A magnetic resonance multi-channel reconstruction method based on deep learning and data self-consistency, comprising the following steps:
[0045] Step 1: Collect multi-channel full-sampling training set K-space data, convert the under-sampling test set K-space data into convoluted images as the input of the reconstruction network, and use the full-sampled K-space data as the training label data for the reconstruction network And generate convolution kernels based on fully sampled K-space data;
[0046] Step 2: After inputting the convoluted image and fusing the under-sampled test set K-space data and convolution kernel, the reconstruction network constructed by repeatedly superimposing SC layer, CNN network and DC layer takes the training label data as the target and backpropagation Train the network parameters to obtain the mapping relationship between the input and output of the reconstructed network;
[0047] Step 3: Input the undersampled test set K-space data in...
Embodiment 2
[0050] Step 1 includes the following steps:
[0051] Step 1.1: Use multi-channel receiving coils to collect full-sampled training set K-space data, and generate under-sampled test set K-space data S through artificial undersampling u , whose size is N x *N y *N c , where N x Represents the collected data S u the number of rows, N y represents the data S u the number of columns, N c Represents the number of receiving channels; manual undersampling does not require manual operation, and can be automated with a variety of algorithms; in the training phase, the undersampling data is generated by discarding some of the full sampling data; in the testing phase, the MRI scanner Undersampling data can be obtained directly; a full sampling data can only have one corresponding undersampling data, so in actual operation, after obtaining a full sampling data, artificial undersampling generates undersampling data on this basis.
[0052] Step 1.2: Undersampling test set K-space data...
PUM
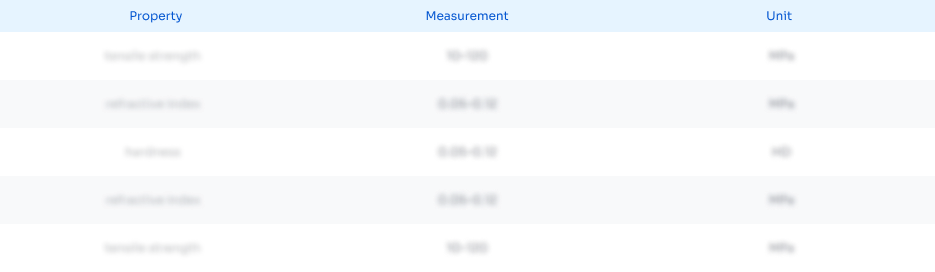
Abstract
Description
Claims
Application Information
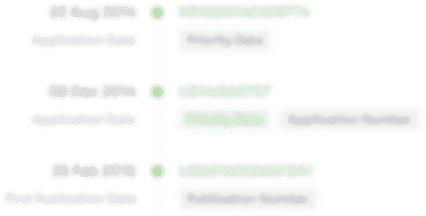
- R&D
- Intellectual Property
- Life Sciences
- Materials
- Tech Scout
- Unparalleled Data Quality
- Higher Quality Content
- 60% Fewer Hallucinations
Browse by: Latest US Patents, China's latest patents, Technical Efficacy Thesaurus, Application Domain, Technology Topic, Popular Technical Reports.
© 2025 PatSnap. All rights reserved.Legal|Privacy policy|Modern Slavery Act Transparency Statement|Sitemap|About US| Contact US: help@patsnap.com