Semi-supervised learning-based twinborn extreme learning machine classification data processing method
An ultra-limited learning machine, semi-supervised learning technology, applied in computer parts, biological neural network models, instruments, etc., can solve the problems of cross data, unable to meet the requirements of generalization ability and computational efficiency at the same time
- Summary
- Abstract
- Description
- Claims
- Application Information
AI Technical Summary
Problems solved by technology
Method used
Image
Examples
Embodiment Construction
[0099] The data processing method of twin extreme learning machine classification based on semi-supervised learning proposed by the present invention is used to learn rules in a small amount of labeled data, and to perform binary classification processing on a large amount of unlabeled data. The method will be described below in conjunction with specific examples , the method includes the following steps:
[0100] (1) Decompose the data set:
[0101] make x i Represents a piece of data in the data set, i=1,2,...,n, n represents the number of data contained in the data set, let the data x i With d features, then x i Represented by a 1×d-dimensional row vector, ie x i =(x i1 ,x i2 ,...,x ij ,...,x id ),x ij represents the data x i The jth feature of , j=1,2,...,d;
[0102] Write all the data in the data set into a matrix X from top to bottom in the form of row vectors:
[0103]
[0104] Divide all the data in the data set into two subsets, namely the subset of labe...
PUM
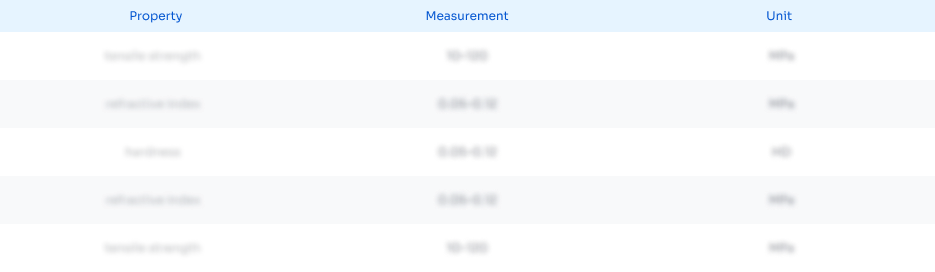
Abstract
Description
Claims
Application Information
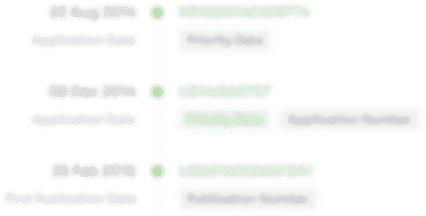
- R&D Engineer
- R&D Manager
- IP Professional
- Industry Leading Data Capabilities
- Powerful AI technology
- Patent DNA Extraction
Browse by: Latest US Patents, China's latest patents, Technical Efficacy Thesaurus, Application Domain, Technology Topic, Popular Technical Reports.
© 2024 PatSnap. All rights reserved.Legal|Privacy policy|Modern Slavery Act Transparency Statement|Sitemap|About US| Contact US: help@patsnap.com