Image classifier adversarial attack defense method based on disturbance evolution
An image classifier and adversarial technology, applied in the direction of instruments, genetic models, genetic rules, etc., can solve problems such as inability to attack defense, inability to directly optimize or compare, and achieve the effect of improving effect, increasing diversity, and increasing quality
- Summary
- Abstract
- Description
- Claims
- Application Information
AI Technical Summary
Problems solved by technology
Method used
Image
Examples
Embodiment Construction
[0053] In order to make the objectives, technical solutions, and advantages of the present invention clearer, the following further describes the present invention in detail with reference to the accompanying drawings and embodiments. It should be understood that the specific embodiments described herein are only used to explain the present invention, and do not limit the protection scope of the present invention.
[0054] This embodiment uses various types of pictures in the ImageNet data set for experiments. Such as Figure 1~3 As shown, the defense method for adversarial attacks on image classifiers based on perturbation evolution provided in this embodiment is divided into three stages, namely, the best confrontation sample generation stage, the confrontation sample detector obtaining stage, and the detection image classification stage. The specific process of each stage is as follows:
[0055] The best adversarial example generation stage
[0056] S101: Input the normal pictu...
PUM
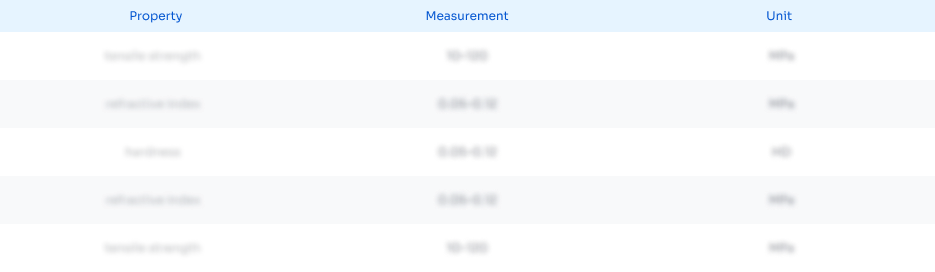
Abstract
Description
Claims
Application Information
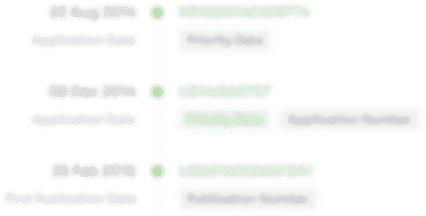
- R&D Engineer
- R&D Manager
- IP Professional
- Industry Leading Data Capabilities
- Powerful AI technology
- Patent DNA Extraction
Browse by: Latest US Patents, China's latest patents, Technical Efficacy Thesaurus, Application Domain, Technology Topic, Popular Technical Reports.
© 2024 PatSnap. All rights reserved.Legal|Privacy policy|Modern Slavery Act Transparency Statement|Sitemap|About US| Contact US: help@patsnap.com