Cloud computation resource scheduling method oriented to distributed machine learning
A machine learning and resource scheduling technology, applied in the software field, can solve the problems of ignoring the quality of model training tasks, large overhead, etc., and achieve the effect of quickly adapting to dynamic changes and improving resource utilization.
- Summary
- Abstract
- Description
- Claims
- Application Information
AI Technical Summary
Problems solved by technology
Method used
Image
Examples
Embodiment Construction
[0016] Below in conjunction with specific embodiment and accompanying drawing, the present invention is described in detail, as figure 1 As shown, the method flow of the embodiment of the present invention:
[0017] Establish a resource scheduling framework such as figure 1 As shown, the scheduler is used to coordinate the resource allocation of multiple machine learning model training jobs sharing cloud computing resources. The job driver contains iterative training logic, generates tasks for each iteration, and tracks the overall progress of the job. The scheduler communicates with drivers that execute jobs concurrently, tracks job progress, and periodically updates resource allocations. At the beginning of each scheduling phase, the scheduler allocates resources based on the job's workload, resource requirements, and task progress. Each job consists of a set of tasks, and each task processes data on a dataset partition. In machine learning model training, tasks update...
PUM
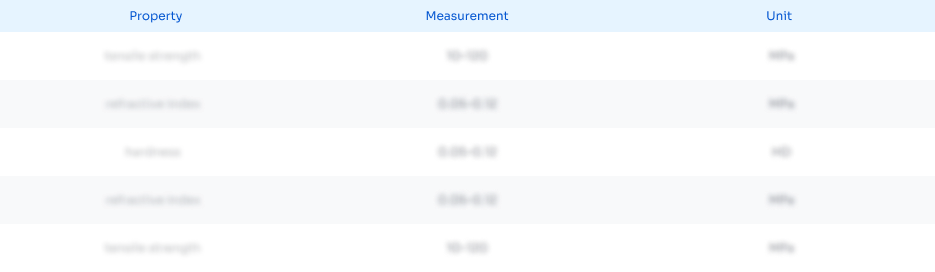
Abstract
Description
Claims
Application Information
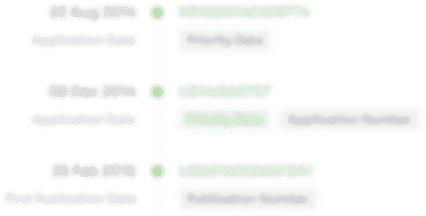
- R&D Engineer
- R&D Manager
- IP Professional
- Industry Leading Data Capabilities
- Powerful AI technology
- Patent DNA Extraction
Browse by: Latest US Patents, China's latest patents, Technical Efficacy Thesaurus, Application Domain, Technology Topic, Popular Technical Reports.
© 2024 PatSnap. All rights reserved.Legal|Privacy policy|Modern Slavery Act Transparency Statement|Sitemap|About US| Contact US: help@patsnap.com