Precise synaptic adjustment method for multi-pulse neural network supervised learning
A neural network and supervised learning technology, applied in the field of spiking neural networks, can solve problems such as difficult to guarantee synaptic weights, low learning efficiency, and difficult to control convergence speed
- Summary
- Abstract
- Description
- Claims
- Application Information
AI Technical Summary
Problems solved by technology
Method used
Image
Examples
Embodiment Construction
[0056] Below in conjunction with accompanying drawing, the present invention is further described:
[0057] Step 1: Use LIF neurons to simulate membrane voltage and synaptic current
[0058] like figure 1 As shown, in the LIF neuron circuit, when the current I continues to increase, the voltage across the capacitor C will break through the threshold V th , at this time, a pulse signal will be excited and transmitted to the next layer of neurons. The formula for the total current in the circuit is:
[0059]
[0060] Set the time as a constant T, and let T=RC, then the above formula can be transformed into:
[0061]
[0062] It can be seen that this circuit is a parallel circuit, and the voltage V(t) on both sides of the capacitor C is exactly the membrane voltage. When the voltage is at t=t f reaches the threshold when V th , a pulse excitation will be generated; after that, the voltage will be quickly reset to the initial value, and a new round of accumulation will...
PUM
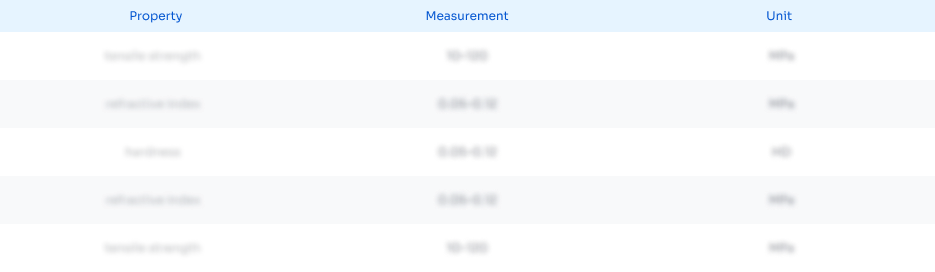
Abstract
Description
Claims
Application Information
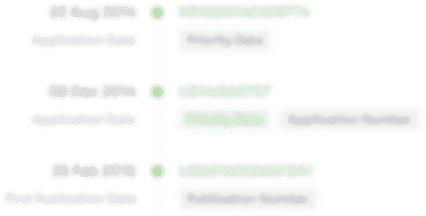
- R&D Engineer
- R&D Manager
- IP Professional
- Industry Leading Data Capabilities
- Powerful AI technology
- Patent DNA Extraction
Browse by: Latest US Patents, China's latest patents, Technical Efficacy Thesaurus, Application Domain, Technology Topic, Popular Technical Reports.
© 2024 PatSnap. All rights reserved.Legal|Privacy policy|Modern Slavery Act Transparency Statement|Sitemap|About US| Contact US: help@patsnap.com