Multi-carrier cognition NOMA resource allocation method based on deep learning
A resource allocation and deep learning technology, which is applied to the separation device of the transmission path, the sub-channel allocation of the transmission path, and the multiple use of the transmission path. problems, to achieve the effect of ensuring energy efficiency and improving spectrum efficiency
- Summary
- Abstract
- Description
- Claims
- Application Information
AI Technical Summary
Problems solved by technology
Method used
Image
Examples
Embodiment Construction
[0070] The present invention will be further described in detail below in conjunction with the accompanying drawings and through specific embodiments. The following embodiments are only descriptive, not restrictive, and cannot limit the protection scope of the present invention.
[0071] In order to achieve the purpose and effect of the technical means, creation features, work flow, and use method of the present invention, and to make the evaluation method easy to understand, the present invention will be further described below in conjunction with specific examples.
[0072] Such as figure 1 As shown, a deep learning-based multi-carrier cognitive NOMA resource allocation method includes the following steps:
[0073] Step 1: Establish a multi-carrier cognitive NOMA system scenario model:
[0074] Combining the single-carrier cognitive NOMA system with the NOMA system based on multi-carrier Orthogonal Frequency Division Multiplexing (OFDM) modulation (ie multi-carrier OFDM-NO...
PUM
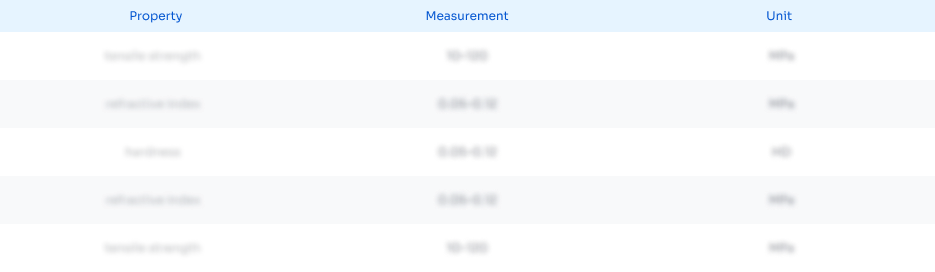
Abstract
Description
Claims
Application Information
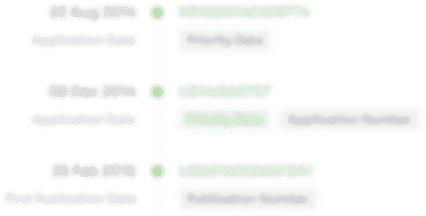
- R&D
- Intellectual Property
- Life Sciences
- Materials
- Tech Scout
- Unparalleled Data Quality
- Higher Quality Content
- 60% Fewer Hallucinations
Browse by: Latest US Patents, China's latest patents, Technical Efficacy Thesaurus, Application Domain, Technology Topic, Popular Technical Reports.
© 2025 PatSnap. All rights reserved.Legal|Privacy policy|Modern Slavery Act Transparency Statement|Sitemap|About US| Contact US: help@patsnap.com