Soft measurement modeling method based on semi-supervised ensemble learning
A technology that integrates learning and modeling methods, applied in character and pattern recognition, instruments, computer components, etc., can solve the problems of inaccurate prediction of key variables and low performance of model prediction, so as to improve product quality and reduce production costs Effect
- Summary
- Abstract
- Description
- Claims
- Application Information
AI Technical Summary
Problems solved by technology
Method used
Image
Examples
Embodiment 1
[0032] This embodiment combines a common chemical process-the debutanizer process as an example, see figure 1 The experimental data comes from the process of the debutanizer E, and the soft sensor modeling method based on semi-supervised integrated learning provided by the present invention is used to predict the butane concentration:
[0033] Step 1: The collection process has label sample set L={X L ,Y L }, L means labeled; and unlabeled sample set U={X U }, U means unlabeled, and for the unlabeled sample set U, the Bagging algorithm is used to generate three unlabeled sample subsets U 1 , U 2 , U 3 .
[0034] Step 2: Use a subset of labeled samples to establish an initial GPR model, f i =GPR(L i ), the initial subset of labeled samples L i =L,i=1, 2, 3.
[0035] Step 3: For U 1 Each sample in x u , Using the nearest neighbor method from L 2 And L 3 Select num samples that are close to each other to obtain the nearest neighbor sample set Ω 2 And Ω 3 .
[0036] Step 4: Use f as in for...
PUM
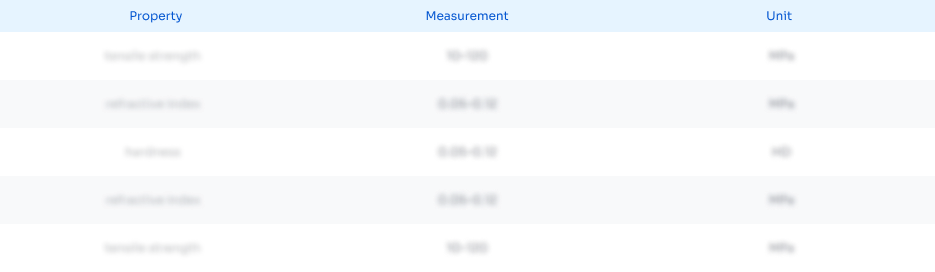
Abstract
Description
Claims
Application Information
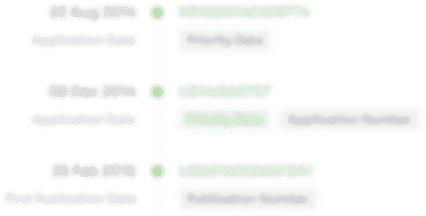
- R&D Engineer
- R&D Manager
- IP Professional
- Industry Leading Data Capabilities
- Powerful AI technology
- Patent DNA Extraction
Browse by: Latest US Patents, China's latest patents, Technical Efficacy Thesaurus, Application Domain, Technology Topic, Popular Technical Reports.
© 2024 PatSnap. All rights reserved.Legal|Privacy policy|Modern Slavery Act Transparency Statement|Sitemap|About US| Contact US: help@patsnap.com