Multiscale deep reinforcement machine learning for n-dimensional segmentation in medical imaging
A technology of machine learning and machine learning models, applied in the field of multi-scale deep reinforcement machine learning for N-dimensional segmentation in medical imaging
- Summary
- Abstract
- Description
- Claims
- Application Information
AI Technical Summary
Problems solved by technology
Method used
Image
Examples
Embodiment Construction
[0014] Multiscale deep reinforcement learning generates multiscale deep reinforcement models for N-dimensional (e.g. 3D) segmentation of objects, where N is an integer greater than 1. In this context, segmentation is formulated as learning an image-driven policy for shape evolution that converges to object boundaries. This segmentation is treated as a reinforcement learning problem, and scale-space theory is used to achieve robust and efficient multi-scale shape estimation. The learning challenge of end-to-end regression systems can be addressed by learning an iterative strategy to find segmentations.
[0015] Although trained as a full segmentation method, the trained policy can be used instead or also for shape refinement as a post-processing step. Any segmentation method provides an initial segmentation. Assuming the original segmentation is used as the initial segmentation in a multi-scale deep reinforcement machine learning model, a machine learning strategy is used to ...
PUM
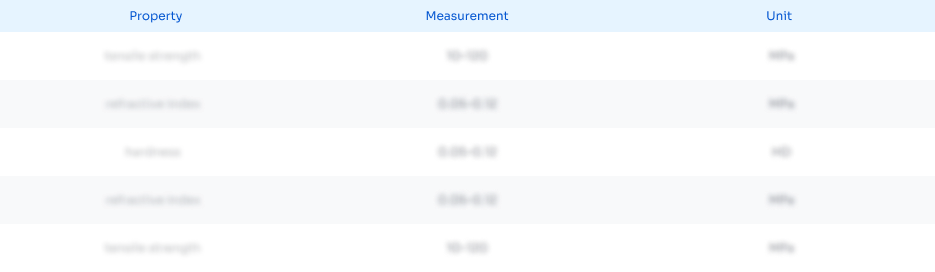
Abstract
Description
Claims
Application Information
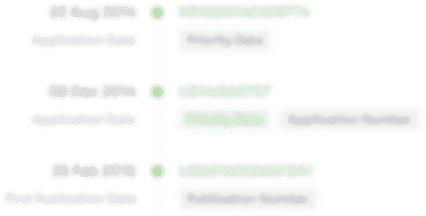
- R&D
- Intellectual Property
- Life Sciences
- Materials
- Tech Scout
- Unparalleled Data Quality
- Higher Quality Content
- 60% Fewer Hallucinations
Browse by: Latest US Patents, China's latest patents, Technical Efficacy Thesaurus, Application Domain, Technology Topic, Popular Technical Reports.
© 2025 PatSnap. All rights reserved.Legal|Privacy policy|Modern Slavery Act Transparency Statement|Sitemap|About US| Contact US: help@patsnap.com