Strip steel surface defect classification method based on combination of statistical features and image features
A technology of statistical features and image features, applied in image analysis, image enhancement, image data processing, etc., to improve the accuracy of classification
- Summary
- Abstract
- Description
- Claims
- Application Information
AI Technical Summary
Problems solved by technology
Method used
Image
Examples
Embodiment Construction
[0022] The present invention will be further described in detail below in conjunction with the accompanying drawings and embodiments.
[0023] The traditional classification of steel strip surface defects is mainly achieved by manually setting rules for the statistical characteristics of defects. For example, for punching defects, it is usually possible to set such as circularity<1.1, average gray value<40, and width<50mm. rule. However, for defects that are difficult to describe specific characteristics, such as phosphorous spots, warped skin, etc., it becomes quite difficult to manually formulate rules. With the advancement of machine learning technology, at this stage, the method of sample self-learning is used to classify defects, that is, a set of manually marked samples of classification results is given, and then machine learning technology is used to allow the computer to automatically find such classification rules. Generally, defects are described by their statistic...
PUM
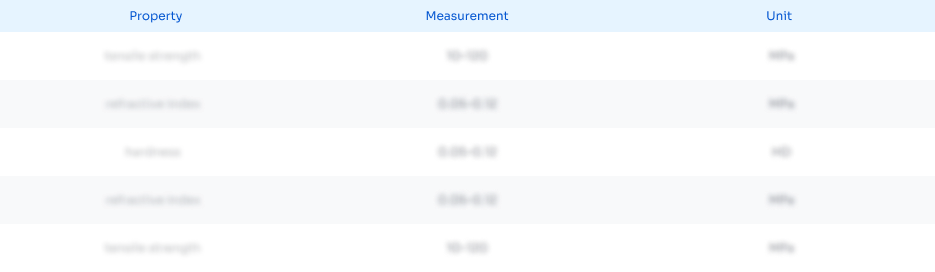
Abstract
Description
Claims
Application Information
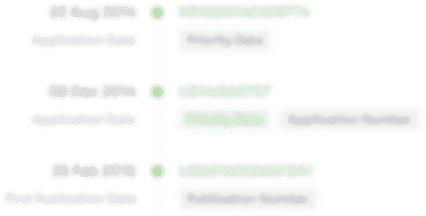
- R&D Engineer
- R&D Manager
- IP Professional
- Industry Leading Data Capabilities
- Powerful AI technology
- Patent DNA Extraction
Browse by: Latest US Patents, China's latest patents, Technical Efficacy Thesaurus, Application Domain, Technology Topic.
© 2024 PatSnap. All rights reserved.Legal|Privacy policy|Modern Slavery Act Transparency Statement|Sitemap