Short-term electric load prediction method based on improved genetic algorithm for optimizing extreme learning machine
An improved genetic algorithm and short-term power load technology, applied in the field of short-term power load forecasting, can solve problems such as network instability
- Summary
- Abstract
- Description
- Claims
- Application Information
AI Technical Summary
Problems solved by technology
Method used
Image
Examples
Embodiment
[0057] Embodiment: Use MATLAB to use the collected active load data and related influencing factors of the main transformer of Zhengzhou City, Henan Province, to carry out experimental verification, and compare and analyze with the prediction results of BP network and ELM network. The specific steps are as follows:
[0058] A. Selection of the input and output of the forecast network model:
[0059] The power load has its own changing rules and is disturbed by other factors such as weather, date type, etc. When performing load forecasting, it is an important part to obtain accurate forecasting by comprehensively considering the fluctuation of the load itself and the disturbance of related factors.
[0060] According to the analysis of short-term power load characteristics, it can be known that the load changes regularly according to the day or week. Since the four seasons are distinct in the Central Plains, the load fluctuation is greatly affected by the weather, and various we...
PUM
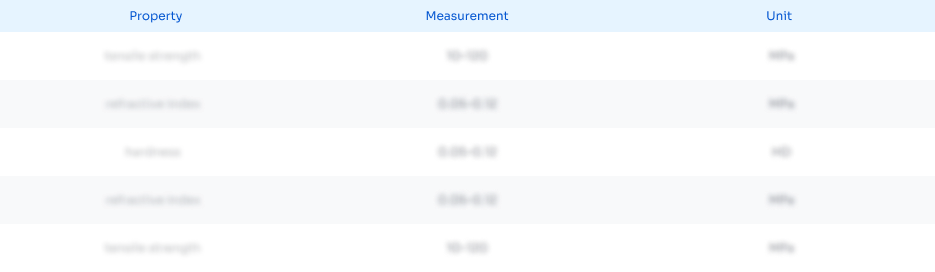
Abstract
Description
Claims
Application Information
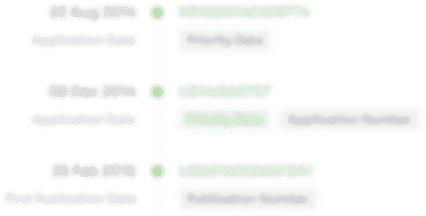
- R&D
- Intellectual Property
- Life Sciences
- Materials
- Tech Scout
- Unparalleled Data Quality
- Higher Quality Content
- 60% Fewer Hallucinations
Browse by: Latest US Patents, China's latest patents, Technical Efficacy Thesaurus, Application Domain, Technology Topic, Popular Technical Reports.
© 2025 PatSnap. All rights reserved.Legal|Privacy policy|Modern Slavery Act Transparency Statement|Sitemap|About US| Contact US: help@patsnap.com