Traffic flow prediction method based on stacked auto-encoder-support vector regression
A technology of support vector regression and self-encoder, applied in traffic flow detection, traffic control system of road vehicles, prediction and other directions, can solve the problems of easy to fall into local optimization, low prediction accuracy, slow convergence speed, etc. Clear, improve prediction accuracy, prevent the effect of local optimization
- Summary
- Abstract
- Description
- Claims
- Application Information
AI Technical Summary
Problems solved by technology
Method used
Image
Examples
Embodiment Construction
[0041] The present invention will be described in further detail below in conjunction with the examples, but the protection scope of the present invention is not limited thereto.
[0042] The invention relates to a traffic flow prediction method based on stack autoencoder-support vector regression, and the method includes the following steps.
[0043] Step 1: Build a stacked autoencoder-support vector regression combination model.
[0044] In the step 1, the stacked autoencoder of the stacked autoencoder-support vector regression combined model includes N stacked autoencoders, N≥2; any of the autoencoders includes 1 input layer, 1 hidden layer and 1 output layer, the stack autoencoder as a whole includes 1 input layer and 1 output layer.
[0045] In the present invention, the autoencoder is a neural network model, and the basic autoencoder model can be regarded as a three-layer neural network structure, which is composed of an input layer, a hidden layer and an output layer. ...
PUM
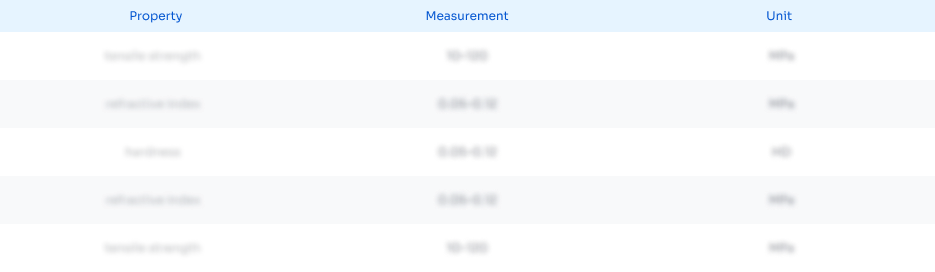
Abstract
Description
Claims
Application Information
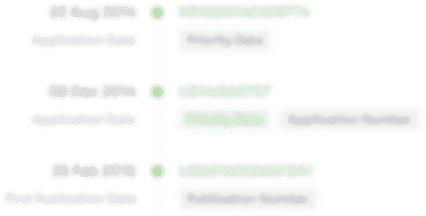
- R&D Engineer
- R&D Manager
- IP Professional
- Industry Leading Data Capabilities
- Powerful AI technology
- Patent DNA Extraction
Browse by: Latest US Patents, China's latest patents, Technical Efficacy Thesaurus, Application Domain, Technology Topic, Popular Technical Reports.
© 2024 PatSnap. All rights reserved.Legal|Privacy policy|Modern Slavery Act Transparency Statement|Sitemap|About US| Contact US: help@patsnap.com