Residual depth feature target tracking method for drift detection
A deep feature and target tracking technology, which is applied in the fields of image processing and computer vision, can solve the problems that the tracking speed cannot meet the needs of real-time tracking, the real-time tracking performance can not meet the requirements well, and unfavorable target tracking and other problems
- Summary
- Abstract
- Description
- Claims
- Application Information
AI Technical Summary
Problems solved by technology
Method used
Image
Examples
Embodiment Construction
[0064] In order to make the object, technical solution and advantages of the present invention clearer, the present invention will be described in detail below with reference to the accompanying drawings and specific embodiments.
[0065] Such as Figure 4 It is a method frame diagram of the present invention, and concrete steps are as follows:
[0066] (1) Training residual deep feature network
[0067] For the entire network structure see figure 1 , containing 4 convolutional layers, 2 fully connected layers and 1 residual structure. The specific operations included in Conv1 are convolutional layer → BN (Batch Normalization) layer → pooling layer. The convolution layer contains multiple convolution kernels, which can extract features from different aspects, and these features can distinguish the target most essentially; as the normalization layer of the network, the BN layer can normalize the output of the convolution layer, and can Speed up the training network, preve...
PUM
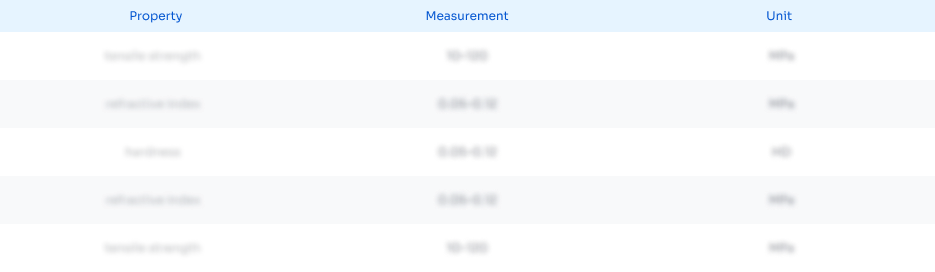
Abstract
Description
Claims
Application Information
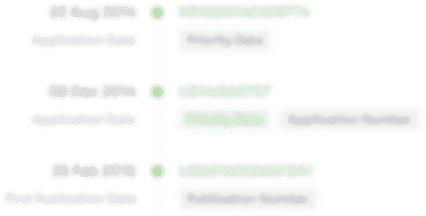
- R&D Engineer
- R&D Manager
- IP Professional
- Industry Leading Data Capabilities
- Powerful AI technology
- Patent DNA Extraction
Browse by: Latest US Patents, China's latest patents, Technical Efficacy Thesaurus, Application Domain, Technology Topic, Popular Technical Reports.
© 2024 PatSnap. All rights reserved.Legal|Privacy policy|Modern Slavery Act Transparency Statement|Sitemap|About US| Contact US: help@patsnap.com