A distance mapping pattern classification method
A technology of mapping patterns and classification methods, applied in character and pattern recognition, instruments, computer parts, etc., can solve the problems of relatively large impact on classification accuracy and neglect of training sample value, and achieve good real-time performance and high classification accuracy. Effect
- Summary
- Abstract
- Description
- Claims
- Application Information
AI Technical Summary
Problems solved by technology
Method used
Image
Examples
Embodiment Construction
[0022] Considering the problems existing in the traditional k-nearest neighbor method, the present invention uses the extracted sample features to calculate the Euclidean distance between the sample to be classified and all the training samples, omits the determination of the k value, and directly determines the mathematical relationship between the Euclidean distance and the classification result through function mapping. relationship, and then propose a distance mapping classifier, that is, the Euclidean distance (independent variable) between the sample to be classified and all training samples is obtained through mathematical calculation (function mapping) to obtain the classification result (dependent variable) of the sample to be classified.
[0023] The invention provides a distance mapping classification method. The basic principle of distance mapping is to map different categories of sample features to different category labels.
[0024] The distance map classifier co...
PUM
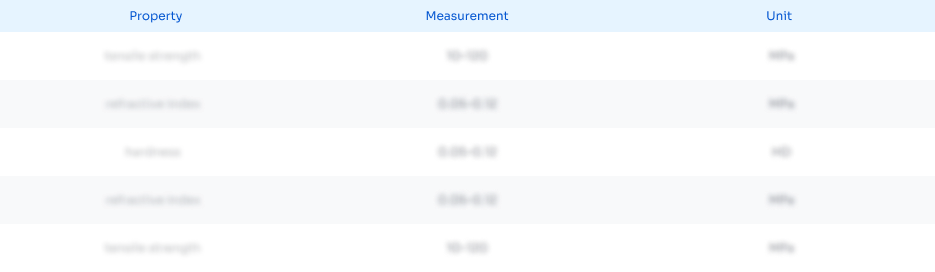
Abstract
Description
Claims
Application Information
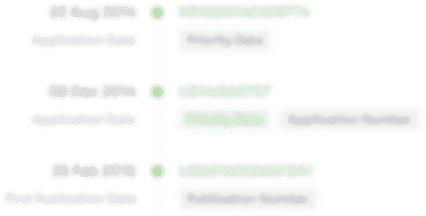
- R&D
- Intellectual Property
- Life Sciences
- Materials
- Tech Scout
- Unparalleled Data Quality
- Higher Quality Content
- 60% Fewer Hallucinations
Browse by: Latest US Patents, China's latest patents, Technical Efficacy Thesaurus, Application Domain, Technology Topic, Popular Technical Reports.
© 2025 PatSnap. All rights reserved.Legal|Privacy policy|Modern Slavery Act Transparency Statement|Sitemap|About US| Contact US: help@patsnap.com