Photovoltaic power interval prediction method combining neural network and parameter estimation
A neural network and parameter estimation technology, which is applied to biological neural network models, predictions, calculations, etc., can solve problems such as poor stability and generalization ability, insufficient precision, and large amount of calculations
- Summary
- Abstract
- Description
- Claims
- Application Information
AI Technical Summary
Problems solved by technology
Method used
Image
Examples
Embodiment Construction
[0064] The present invention proposes a photovoltaic power interval prediction method combined with neural network and parameter estimation, which will be described below in conjunction with the accompanying drawings.
[0065] figure 1 Shown is the photovoltaic power interval prediction method combined with deep recurrent neural network and parameter estimation. The present invention utilizes the long short-term memory network photovoltaic power prediction method to perform short-term photovoltaic power prediction one day in advance, and its eigenvector is [cumulative day, Ambient temperature, ambient humidity, wind speed, solar irradiance], where the accumulated days are recorded as 1 from January 1st, and so on December 31st as 365. The time resolution of the data is one hour. The model goal is to input the photovoltaic power data and weather data at 24 full points every day 30 days before the forecast date, and the forecast model outputs the corresponding photovoltaic powe...
PUM
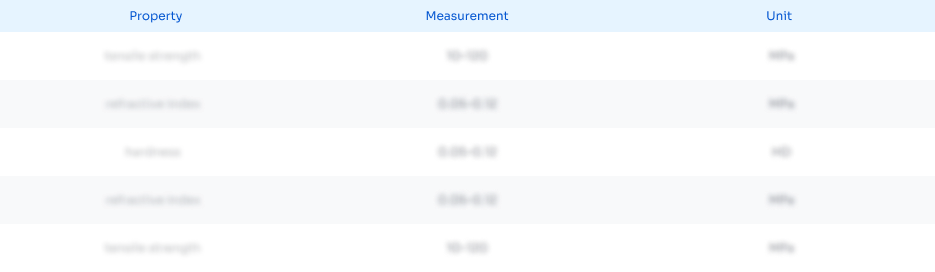
Abstract
Description
Claims
Application Information
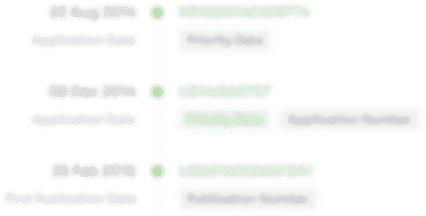
- R&D Engineer
- R&D Manager
- IP Professional
- Industry Leading Data Capabilities
- Powerful AI technology
- Patent DNA Extraction
Browse by: Latest US Patents, China's latest patents, Technical Efficacy Thesaurus, Application Domain, Technology Topic, Popular Technical Reports.
© 2024 PatSnap. All rights reserved.Legal|Privacy policy|Modern Slavery Act Transparency Statement|Sitemap|About US| Contact US: help@patsnap.com