A method of license plate image deblurring based on depth learning
A license plate image and motion blur technology, applied in the field of image processing, can solve the problems of low restoration quality, long time-consuming license plate restoration process, pixel distortion, etc., and achieve good restoration effect
- Summary
- Abstract
- Description
- Claims
- Application Information
AI Technical Summary
Problems solved by technology
Method used
Image
Examples
Embodiment Construction
[0049] The specific implementation of the present invention will be further described below in conjunction with the accompanying drawings.
[0050] figure 1 is a flowchart of the training phase of the generative adversarial network. The fuzzy image set B is input into the generator G to obtain the generated image set L, which is used as the input of the discriminator D to obtain the discrimination result of the discriminator. Similarly, the clear image set S is also used as the input of the discriminator to obtain Discrimination result. The determination result indicates whether the input is determined from the clear image set or the generated image set. If the determination result is >0.5, it is determined as the clear image set S; otherwise, it is determined as the generated image set L. Calculate the error between the judgment result and the real label data, use the gradient descent algorithm to optimize the discriminator, then calculate the mean value of the error betwee...
PUM
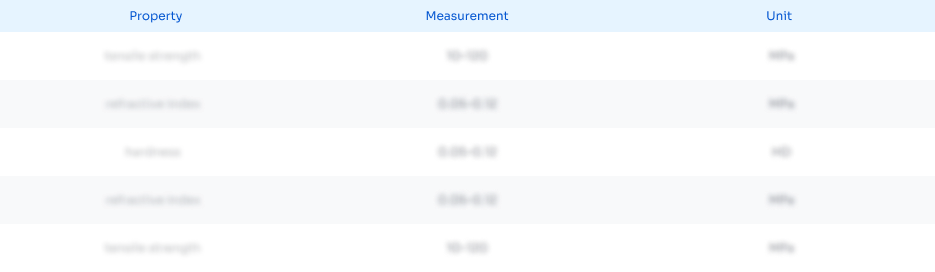
Abstract
Description
Claims
Application Information
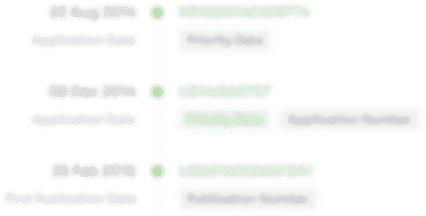
- R&D Engineer
- R&D Manager
- IP Professional
- Industry Leading Data Capabilities
- Powerful AI technology
- Patent DNA Extraction
Browse by: Latest US Patents, China's latest patents, Technical Efficacy Thesaurus, Application Domain, Technology Topic.
© 2024 PatSnap. All rights reserved.Legal|Privacy policy|Modern Slavery Act Transparency Statement|Sitemap