A pedestrian detection method based on depth-supervised learning to extract multi-level features of images
A pedestrian detection and supervised learning technology, applied in the field of pedestrian detection, can solve the problems of limiting the accuracy of the infrared pedestrian detection system, affecting the results of neural network training, and occupying a large amount of memory space, so as to reduce network parameters and computational complexity and increase reusability. , the effect of saving energy
- Summary
- Abstract
- Description
- Claims
- Application Information
AI Technical Summary
Problems solved by technology
Method used
Image
Examples
Embodiment Construction
[0032] A pedestrian detection method for extracting multi-level features of images based on deep supervised learning of the present invention will be described in detail below with reference to embodiments and drawings.
[0033] A kind of pedestrian detection method based on deep supervised learning of the present invention extracts image multi-level feature, comprises the following steps:
[0034] 1) build infrared pedestrian detection training set and test data set; described infrared pedestrian detection training set and test data set required infrared image data adopt Elektra Research Center's CVC-09 and CVC-14 data set, the present invention constructs infrared The pedestrian detection training set and test data set include:
[0035] (1) Modify the annotation data format of the infrared image to the VOC dataset standard;
[0036] (2) Merge the two data sets of CVC-09 and CVC-14;
[0037] (3) Select 12534 pictures as the training set, and the remaining 3600 pictures as t...
PUM
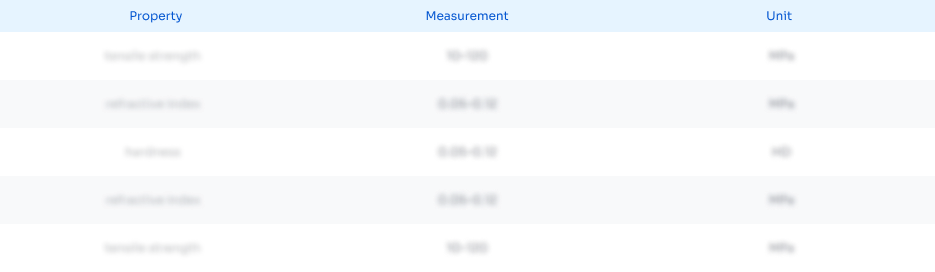
Abstract
Description
Claims
Application Information
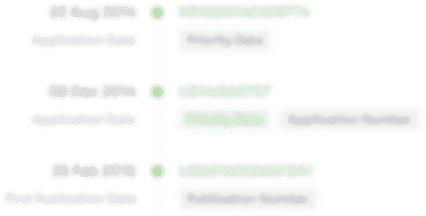
- R&D
- Intellectual Property
- Life Sciences
- Materials
- Tech Scout
- Unparalleled Data Quality
- Higher Quality Content
- 60% Fewer Hallucinations
Browse by: Latest US Patents, China's latest patents, Technical Efficacy Thesaurus, Application Domain, Technology Topic, Popular Technical Reports.
© 2025 PatSnap. All rights reserved.Legal|Privacy policy|Modern Slavery Act Transparency Statement|Sitemap|About US| Contact US: help@patsnap.com