Classification model generation method based on missing data
A missing data, classification model technology, applied in the field of neural networks, can solve the problems of low model accuracy, inability to model training, ignoring the use of high-order relationship label information of features, etc., to achieve the effect of improving accuracy and accuracy
- Summary
- Abstract
- Description
- Claims
- Application Information
AI Technical Summary
Problems solved by technology
Method used
Image
Examples
Embodiment Construction
[0041] The following will clearly and completely describe the technical solutions in the embodiments of the present invention with reference to the accompanying drawings in the embodiments of the present invention. Obviously, the described embodiments are only some, not all, embodiments of the present invention. Based on the embodiments of the present invention, all other embodiments obtained by persons of ordinary skill in the art without creative efforts fall within the protection scope of the present invention.
[0042] refer to figure 1 , is a schematic flowchart of a method for generating a classification model based on missing data provided by an embodiment of the present invention, including S101-S104:
[0043] S101: Acquire missing data feature matrix.
[0044] S102: Express the missing data feature matrix with a probability density function of a Gaussian mixture model to obtain a first feature matrix.
[0045] S103: Obtain labels corresponding to data missing types,...
PUM
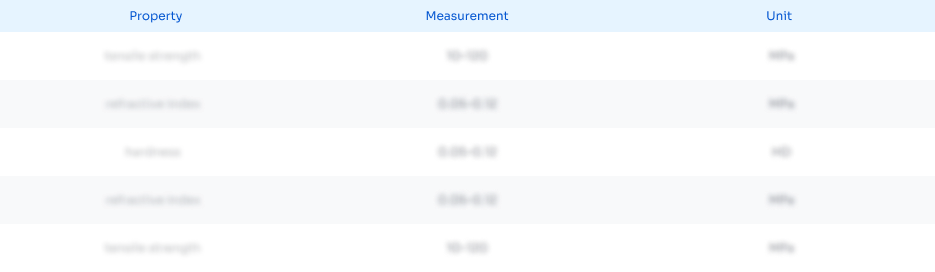
Abstract
Description
Claims
Application Information
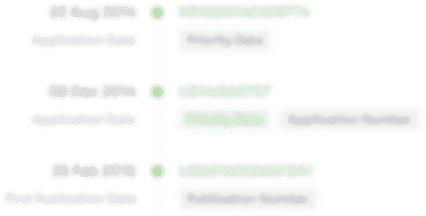
- R&D
- Intellectual Property
- Life Sciences
- Materials
- Tech Scout
- Unparalleled Data Quality
- Higher Quality Content
- 60% Fewer Hallucinations
Browse by: Latest US Patents, China's latest patents, Technical Efficacy Thesaurus, Application Domain, Technology Topic, Popular Technical Reports.
© 2025 PatSnap. All rights reserved.Legal|Privacy policy|Modern Slavery Act Transparency Statement|Sitemap|About US| Contact US: help@patsnap.com