An image classification method based on octal convolution neural network
A convolutional neural network and classification method technology, applied in biological neural network models, neural architectures, instruments, etc., can solve problems such as affecting the accuracy of human behavior recognition, ignoring spatial dependencies, and destroying the color characteristics of real environments.
- Summary
- Abstract
- Description
- Claims
- Application Information
AI Technical Summary
Problems solved by technology
Method used
Image
Examples
Embodiment Construction
[0061] The present invention will be further described in detail below in conjunction with the drawings:
[0062] figure 1 It is a flowchart of the present invention, which mainly includes the following steps:
[0063] Step 1: Input N training images with a size of m×n pixels, preprocess the N training images, and learn the imaginary part of the octon. N, m, and n are positive integers, and N can be divisible by 8. Embed the octonion X into the real value and express the octonion with an eight-order real matrix, and apply the addition and multiplication of the matrix to the addition and multiplication of the octonion.
[0064] First, perform a real batch normalization operation on the input feature map R, and output the data of the i-1th layer of the network O i-1 Expressed as a four-dimensional matrix (m, f, p, q), where m is the size of a batch of data, f is the number of feature maps, p, q are the width and height of the feature maps, if you consider each feature map as It is a f...
PUM
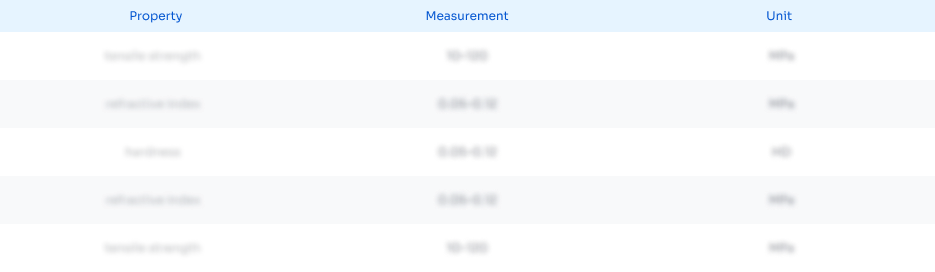
Abstract
Description
Claims
Application Information
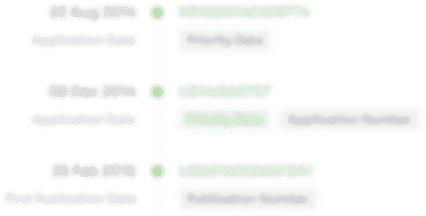
- R&D Engineer
- R&D Manager
- IP Professional
- Industry Leading Data Capabilities
- Powerful AI technology
- Patent DNA Extraction
Browse by: Latest US Patents, China's latest patents, Technical Efficacy Thesaurus, Application Domain, Technology Topic, Popular Technical Reports.
© 2024 PatSnap. All rights reserved.Legal|Privacy policy|Modern Slavery Act Transparency Statement|Sitemap|About US| Contact US: help@patsnap.com