Pedestrian re-recognition method based on multi-layer neural network for cross-scene pedestrian recognition
A multi-layer neural network and pedestrian re-identification technology, which is applied in character and pattern recognition, instruments, computer components, etc., can solve the problem of low recognition accuracy of cross-scene pedestrian re-identification models
- Summary
- Abstract
- Description
- Claims
- Application Information
AI Technical Summary
Problems solved by technology
Method used
Image
Examples
Embodiment Construction
[0064] In order to make the object, technical solution and advantages of the present invention clearer, the present invention will be further described in detail below in combination with specific examples and with reference to the accompanying drawings.
[0065] The overall implementation flow chart of the present invention is as figure 1 As shown, the specific implementation is as follows:
[0066] Step 1. Collect the pedestrian video in the camera under the current scene, and intercept the video frame. This embodiment uses the data set i-LIDS image as the video frame image under the current scene;
[0067] Step 2. Perform feature extraction and dimensionality reduction processing on the images of pedestrians in the video frames of the dataset i-LIDS; the i-LIDS dataset includes 476 images of 119 pedestrians in total. For normalization processing, the pixels of each image are processed into 128×48. In this embodiment, an area block with a pixel size of 16×16 is taken, and e...
PUM
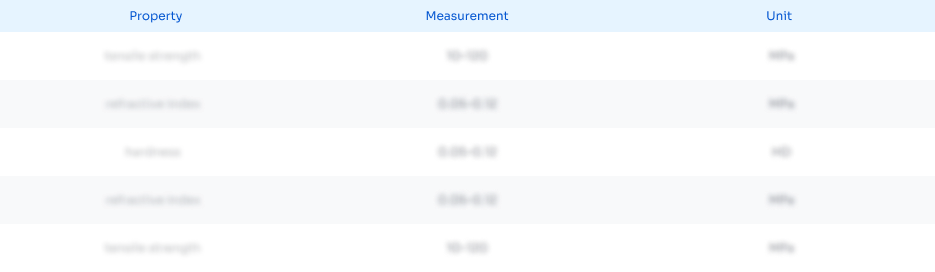
Abstract
Description
Claims
Application Information
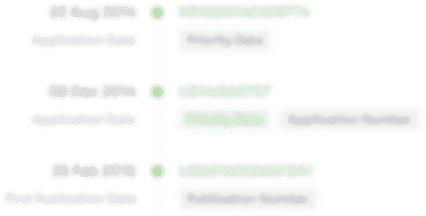
- Generate Ideas
- Intellectual Property
- Life Sciences
- Materials
- Tech Scout
- Unparalleled Data Quality
- Higher Quality Content
- 60% Fewer Hallucinations
Browse by: Latest US Patents, China's latest patents, Technical Efficacy Thesaurus, Application Domain, Technology Topic, Popular Technical Reports.
© 2025 PatSnap. All rights reserved.Legal|Privacy policy|Modern Slavery Act Transparency Statement|Sitemap|About US| Contact US: help@patsnap.com