A Tag Semantic Learning Method Based on Network Structure and Semantic Correlation Measure
A semantic correlation and network structure technology, applied in the field of label semantic learning based on network structure and semantic correlation measurement, can solve problems such as difficult to solve label network collaboration facts, unclear topic boundaries, high noise and changeable information, etc., to achieve Improve modeling generalization, ease of operation, and reduce network noise
- Summary
- Abstract
- Description
- Claims
- Application Information
AI Technical Summary
Problems solved by technology
Method used
Image
Examples
Embodiment Construction
[0037] Embodiments of the present invention will be described in further detail below in conjunction with the accompanying drawings.
[0038]The design idea of the present invention is: mainly based on statistical machine learning theory and text mining technology, using user behavior data to construct a highly reliable label network to learn the pure semantic representation of labels. First, the user behavior facts are used to initialize the label network, and then the label network is reconstructed based on the protocol technology and the improved random walk technology, and the label network is constructed by using the label-related text similarity, and finally the reconstruction-based label network and the label based on text similarity Networks learn label semantic vector representations. The network reconstruction in the present invention can be regarded as an automatic multi-path tag strong topic association discovery filter, which filters out weaker associations and ...
PUM
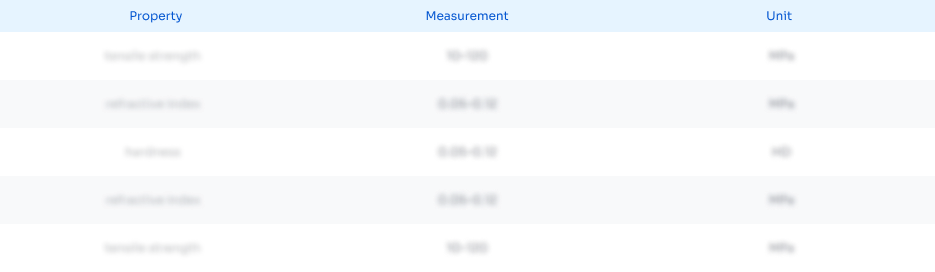
Abstract
Description
Claims
Application Information
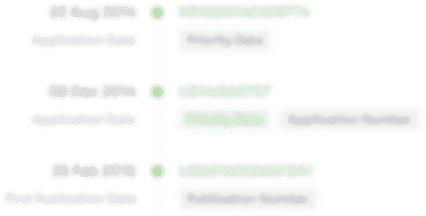
- R&D
- Intellectual Property
- Life Sciences
- Materials
- Tech Scout
- Unparalleled Data Quality
- Higher Quality Content
- 60% Fewer Hallucinations
Browse by: Latest US Patents, China's latest patents, Technical Efficacy Thesaurus, Application Domain, Technology Topic, Popular Technical Reports.
© 2025 PatSnap. All rights reserved.Legal|Privacy policy|Modern Slavery Act Transparency Statement|Sitemap|About US| Contact US: help@patsnap.com