A vegetable identification method and device
A vegetable, gradient descent algorithm technology, applied in the field of image recognition, can solve the problems of labor-intensive, low recognition efficiency, low efficiency, etc., to achieve the effect of improving efficiency
- Summary
- Abstract
- Description
- Claims
- Application Information
AI Technical Summary
Problems solved by technology
Method used
Image
Examples
Embodiment 1
[0084] Please refer to figure 1 , a method for vegetable identification, comprising steps:
[0085] S1. Establish the first convolutional neural network model;
[0086] S2. Collect images of each type of vegetable in different scenarios, and construct corresponding input data sets;
[0087] S3. Perform iterative training on the first convolutional neural network model according to the gradient descent algorithm adaptively updated with the learning rate and the input data set to obtain a second convolutional neural network model. According to the second convolutional neural network Model for vegetable identification;
[0088] In step S3, the gradient descent algorithm adaptively updated according to the learning rate and the input data set are used for iterative training of the first convolutional neural network model, and the second convolutional neural network model is specifically:
[0089] Calculate the value of the loss function of the input data set at the kth iterativ...
Embodiment 2
[0111] This embodiment will further illustrate how the above-mentioned vegetable identification method of the present invention is realized in combination with specific application scenarios:
[0112] 1. Establish the AlexNet model of the convolutional neural network;
[0113] Convolutional neural network models have a variety of models, such as GoogLeNet, ResNet and VGGNet. Compared with other models, the AlexNet model is more mature in theory and has a high recognition rate. The basic composition of the AlexNet model is: 5 convolutional layers, 3 fully connected layers and 1 softmax layer. Among the 5 convolutional layers, the first two convolutional layers and the fifth convolutional layer have pooling layers, and the other two convolutional layers have no pooling layers. In each volume The product layer contains the activation function RELU and local corresponding normalization (LRN) processing, and then undergoes downsampling (pool processing);
[0114] The convolutional...
Embodiment 3
[0146] Please refer to figure 2, a device 1 for vegetable identification, comprising a memory 2, a processor 3 and a computer program stored in the memory 2 and operable on the processor 3, the processor 3 implements the first embodiment when executing the computer program each step.
[0147] In summary, the method and device for vegetable recognition provided by the present invention, by establishing the first convolutional neural network model, and collecting images of each type of vegetable in different scenarios, and constructing corresponding input data sets, according to learning The gradient descent algorithm of rate adaptive update and the input data set iteratively train the first convolutional neural network model to obtain a second convolutional neural network model, and carry out vegetable identification according to the second convolutional neural network model , which improves the efficiency of vegetable recognition, and trains the first convolutional neural ne...
PUM
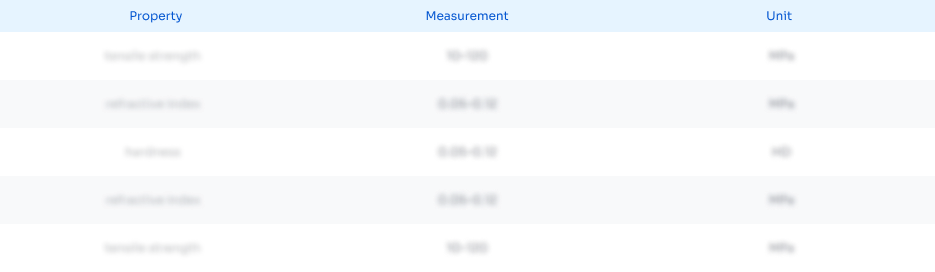
Abstract
Description
Claims
Application Information
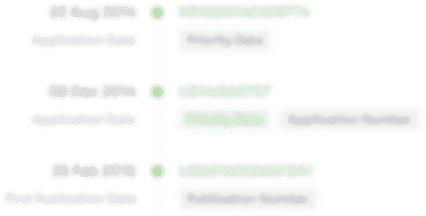
- R&D
- Intellectual Property
- Life Sciences
- Materials
- Tech Scout
- Unparalleled Data Quality
- Higher Quality Content
- 60% Fewer Hallucinations
Browse by: Latest US Patents, China's latest patents, Technical Efficacy Thesaurus, Application Domain, Technology Topic, Popular Technical Reports.
© 2025 PatSnap. All rights reserved.Legal|Privacy policy|Modern Slavery Act Transparency Statement|Sitemap|About US| Contact US: help@patsnap.com