Single frequency point frequency spectrum prediction method based on optimum long short-term memory model
A long-short-term memory and spectrum prediction technology, applied in neural learning methods, biological neural network models, transmission monitoring, etc., can solve unfavorable spectrum state evolution, SVR model lacks structured method model parameters, loss of original data information, etc. problem, to achieve the effect of simplifying the hyperparameter selection problem
- Summary
- Abstract
- Description
- Claims
- Application Information
AI Technical Summary
Problems solved by technology
Method used
Image
Examples
Embodiment 1
[0082] A specific embodiment of the present invention is described as follows, the system emulation adopts keras platform and python language, and the spectrum data adopts the frequency points of the GSM1800 downlink frequency band in the spectrum measurement activities of the University of Aachen, Germany. This embodiment verifies the stability and superiority of proposed model and method ( image 3 and Figure 4 ). Taking the frequency point of 1863.6MHz as an example, 8-level quantization is first performed during preprocessing, and mapped to the interval [-1,1] according to formula (1). When constructing the data set, the window size is set to 120, the time span is about 3.5s, and the training set and test set are divided according to the ratio of 4:1. The batch size during training is 150, and the total number of iterations is 100, which can ensure convergence. The following five factors are considered when determining the hyperparameters of the deep learning model, an...
PUM
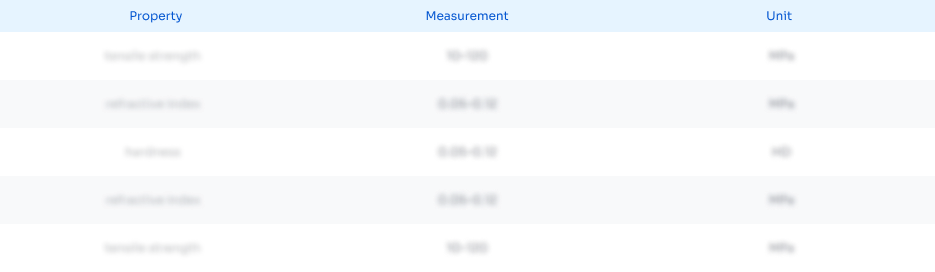
Abstract
Description
Claims
Application Information
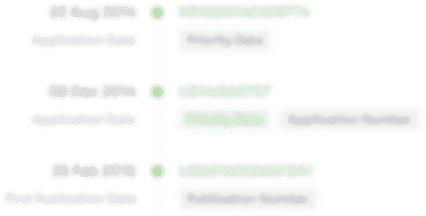
- R&D
- Intellectual Property
- Life Sciences
- Materials
- Tech Scout
- Unparalleled Data Quality
- Higher Quality Content
- 60% Fewer Hallucinations
Browse by: Latest US Patents, China's latest patents, Technical Efficacy Thesaurus, Application Domain, Technology Topic, Popular Technical Reports.
© 2025 PatSnap. All rights reserved.Legal|Privacy policy|Modern Slavery Act Transparency Statement|Sitemap|About US| Contact US: help@patsnap.com