Method for diagnosing HVAC (heating, ventilation and air conditioning) system gradual failure based on deep learning
A deep learning and fault diagnosis technology, applied in general control systems, control/regulation systems, testing/monitoring control systems, etc., can solve problems such as high dependence on fault data, inability to predict the development trend of gradual faults, and inability to accurately measure systems. , to reduce the difficulty of data acquisition, achieve high-precision diagnosis, and facilitate maintenance time.
- Summary
- Abstract
- Description
- Claims
- Application Information
AI Technical Summary
Problems solved by technology
Method used
Image
Examples
Embodiment approach
[0040] In order to achieve accurate modeling of the system, we use a large amount of health system data to train the RNN model. Since there is basically no gradual failure in the initial stage of the HVAC system, a large amount of health data can be obtained. The specific training steps are as follows:
[0041] 2.1) First select the input and output parameters for model training. Among them, the output parameters are the target parameters of the failure, and the input parameters are all system parameters that will affect the target parameters.
[0042] 2.2) Organize the health system data set collected in advance, extract the input parameter data as the input data set, and extract the output parameter data as the output data set.
[0043] 2.3) Use input and output data sets to train the RNN model to obtain a system model for the fault.
[0044] Because the RNN structure is complex enough, when the amount of data is large enough, we can get a model with high enough accuracy. This pro...
PUM
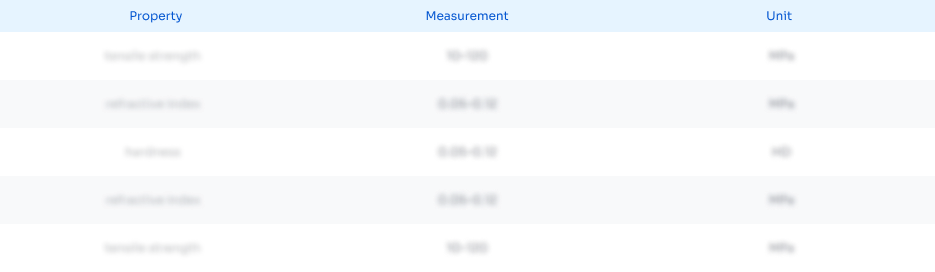
Abstract
Description
Claims
Application Information
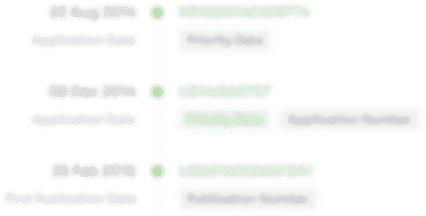
- R&D Engineer
- R&D Manager
- IP Professional
- Industry Leading Data Capabilities
- Powerful AI technology
- Patent DNA Extraction
Browse by: Latest US Patents, China's latest patents, Technical Efficacy Thesaurus, Application Domain, Technology Topic, Popular Technical Reports.
© 2024 PatSnap. All rights reserved.Legal|Privacy policy|Modern Slavery Act Transparency Statement|Sitemap|About US| Contact US: help@patsnap.com