Neural network weight discretization method and system
A neural network and discretization technology, applied in the field of neural network weight discretization, to achieve the effect of reducing computational complexity and storage space consumption
- Summary
- Abstract
- Description
- Claims
- Application Information
AI Technical Summary
Problems solved by technology
Method used
Image
Examples
Embodiment Construction
[0069] In order to make the object, technical solution and advantages of the present invention clearer, the present invention will be further described in detail below in conjunction with the accompanying drawings and embodiments. It should be understood that the specific embodiments described here are only used to explain the present invention, not to limit the present invention.
[0070] The main operation part of the neural network calculation is the multiplication and accumulation operation of the input data and the weight, and the accuracy of the weight directly affects the complexity of the calculation. The present invention can effectively reduce the accuracy requirement of the weight through the method of probability sampling, significantly reduce the memory usage of the weight memory and reduce the operation complexity under the premise of almost no influence on the performance. This method can be used not only for offline learning of neural networks, but also for on-...
PUM
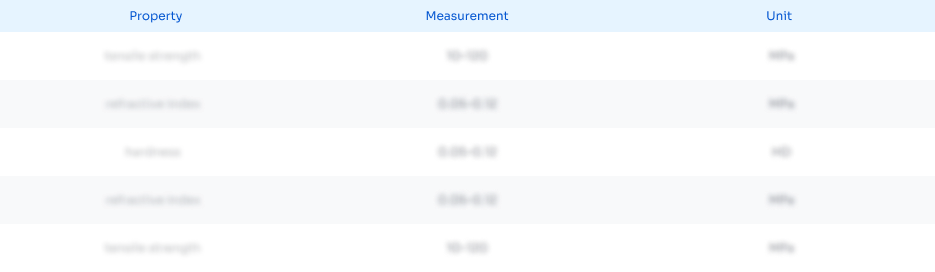
Abstract
Description
Claims
Application Information
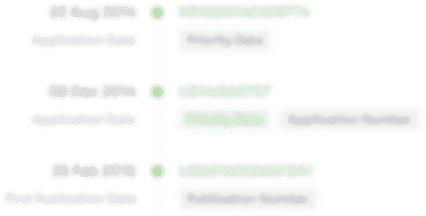
- R&D
- Intellectual Property
- Life Sciences
- Materials
- Tech Scout
- Unparalleled Data Quality
- Higher Quality Content
- 60% Fewer Hallucinations
Browse by: Latest US Patents, China's latest patents, Technical Efficacy Thesaurus, Application Domain, Technology Topic, Popular Technical Reports.
© 2025 PatSnap. All rights reserved.Legal|Privacy policy|Modern Slavery Act Transparency Statement|Sitemap|About US| Contact US: help@patsnap.com