An image processing method for expanding a data set under a small sample
An image processing and sample image technology, applied in the field of image processing, can solve the problems of increasing difficulty in image recognition and inability to recognize, and achieve the effect of increasing a reasonable number of samples and high recognition accuracy
- Summary
- Abstract
- Description
- Claims
- Application Information
AI Technical Summary
Problems solved by technology
Method used
Image
Examples
Embodiment 1
[0032] An image processing method for expanding data sets under small samples, including small sample sets, further comprising the following steps:
[0033] S1. Classify the samples in the small sample set;
[0034] S2. For the classification in step S1, formulate an image transformation strategy for each category;
[0035] S3. Transform the samples in the small sample set according to the transformation strategy formulated in step S2 to obtain virtual samples, and add both the virtual samples and the original samples to the large sample set to realize the expansion of the sample set.
[0036] In order to solve the problem of poor recognition accuracy of small-sample images, the present invention adopts sample expansion technology to overcome the problems caused by insufficient samples, and provides a more general image processing method. This method translates, rotates, mirrors, zooms, perspectives, transforms contrast and brightness, adds noise, and blurs the original train...
Embodiment 2
[0038] The difference between this embodiment and Embodiment 1 is that further, the classification criteria in step S1 include:
[0039] S101: Determine whether the sample image includes the target image, and classify the sample image into two types: images including the target and images not including the target;
[0040] S102: Carry out feature judgment on the images classified in step S101, determine the proportion of the feature image in the sample image, and classify again the images including the target and the images not including the target according to the proportion.
[0041] Further, the transformation strategy of the image in the step S2 includes:
[0042] S201, setting the number of transformations: controlling the number of extensions by setting the sampling rate for each transformation, and controlling the number of virtual samples generated by the transformation according to the requirements of different training tasks;
[0043] S202. Setting the degree of tra...
Embodiment 3
[0050] Let's take the samples required for training to recognize a driver's phone call as an example. All sample image data are 32*32 in size, and the samples in the training set are divided into four categories according to the specific characteristics of the samples, and customized image transformation processing is performed respectively.
[0051] S103 indicates that among the heads of people making calls, the size of the head is relatively small, that is, there is room for panning around the head, and the ear or mobile phone will not be panned out of the picture.
[0052] S104 indicates that among the heads of the people making the call, the size of the head is relatively large, that is, there is almost no extra space for translation around the head.
[0053] S105 indicates that among the heads of people who did not make a call, the size of the head is relatively small;
[0054] S106 indicates that among the heads of the people who did not make a call, the head size is re...
PUM
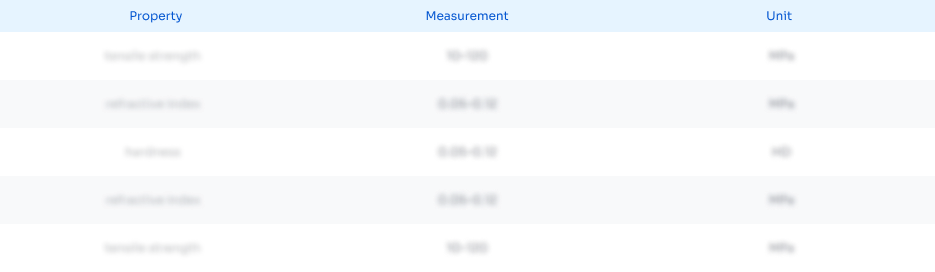
Abstract
Description
Claims
Application Information
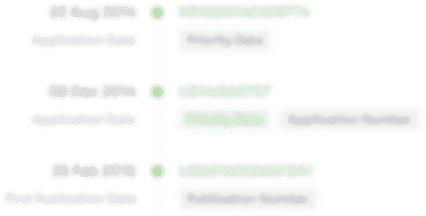
- R&D Engineer
- R&D Manager
- IP Professional
- Industry Leading Data Capabilities
- Powerful AI technology
- Patent DNA Extraction
Browse by: Latest US Patents, China's latest patents, Technical Efficacy Thesaurus, Application Domain, Technology Topic, Popular Technical Reports.
© 2024 PatSnap. All rights reserved.Legal|Privacy policy|Modern Slavery Act Transparency Statement|Sitemap|About US| Contact US: help@patsnap.com