Traffic lane detection method and device based on depth learning multitask network
A lane line detection and deep learning technology, applied in the field of intelligent video surveillance, can solve problems such as image adaptability defects, reduce detection accuracy, loss of edge details, etc., achieve adaptability and accuracy improvement, meet real-time requirements, adapt to strong effect
- Summary
- Abstract
- Description
- Claims
- Application Information
AI Technical Summary
Problems solved by technology
Method used
Image
Examples
Embodiment Construction
[0044] It should be noted that, in the case of no conflict, the embodiments of the present invention and the features in the embodiments can be combined with each other.
[0045] The new method for detecting lane lines in traffic scenes based on deep learning multi-task network of the present invention is realized in the following ways:
[0046] When extracting brightness and edge information, based on the spatial correlation of pixels in the actual scene image, the image is first down-sampled with a sampling rate of 1 / (3*3), that is, the center point is collected every 3 rows and 3 columns. If the original image size If it is W*H, the size of the thumbnail after sampling is (W / 3)*(H / 3), and the edge does not meet 3*3 for padding processing, and the average brightness value is calculated: Then perform two-dimensional convolution on the image to extract edge information. The convolution operator is optimized based on the scharr operator, which enhances the correlation of adjac...
PUM
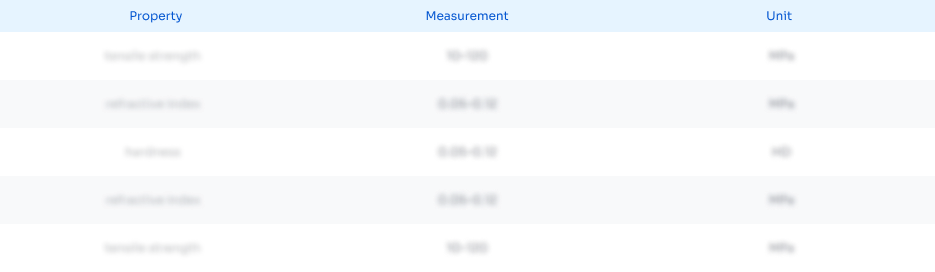
Abstract
Description
Claims
Application Information
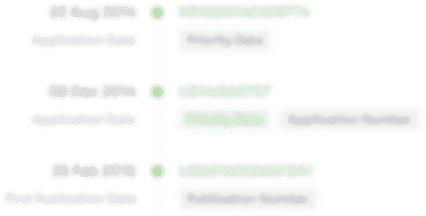
- R&D Engineer
- R&D Manager
- IP Professional
- Industry Leading Data Capabilities
- Powerful AI technology
- Patent DNA Extraction
Browse by: Latest US Patents, China's latest patents, Technical Efficacy Thesaurus, Application Domain, Technology Topic, Popular Technical Reports.
© 2024 PatSnap. All rights reserved.Legal|Privacy policy|Modern Slavery Act Transparency Statement|Sitemap|About US| Contact US: help@patsnap.com