Control method and control device for realizing disease prediction based on eigenvector
A control method and eigenvector technology, which is applied in the fields of case quality control, medical guidance, and medical clinical auxiliary diagnosis, and can solve problems such as lack of comprehensiveness, low efficiency of artificial rules, inaccurate judgment results, etc.
- Summary
- Abstract
- Description
- Claims
- Application Information
AI Technical Summary
Problems solved by technology
Method used
Image
Examples
Embodiment Construction
[0063] In order to better clearly express the technical solution of the present invention, the present invention will be further described below in conjunction with the accompanying drawings.
[0064] figure 1 Showing a specific embodiment of the present invention, a specific flow chart of a control method for realizing disease prediction based on eigenvectors, specifically, including the following steps:
[0065] First, enter step S101, based on the Embedding model, convert one or more original vectorized representations into dense vectorized representations, and vectorize the representation of words, the abstraction of entities into mathematical descriptions, and modeling can be applied to many In tasks, such as comparing the similarity between words and words, it can be directly determined by the cosine distance measurement between vectors. The word vectorization representation needs to obtain the features in the text data based on text data, etc., for each character in the...
PUM
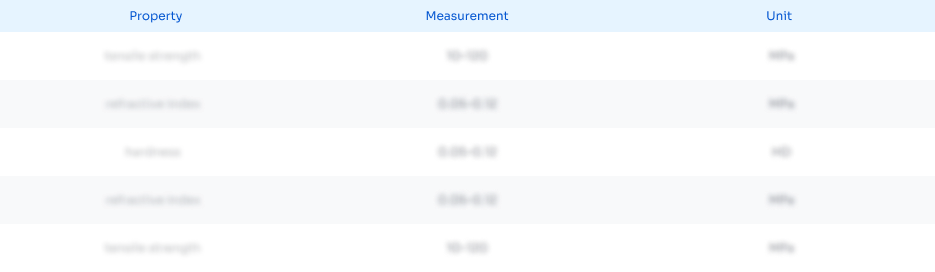
Abstract
Description
Claims
Application Information
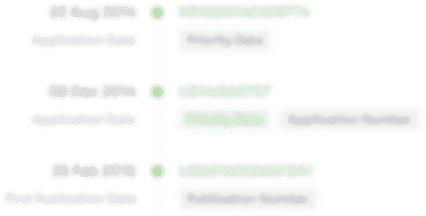
- R&D Engineer
- R&D Manager
- IP Professional
- Industry Leading Data Capabilities
- Powerful AI technology
- Patent DNA Extraction
Browse by: Latest US Patents, China's latest patents, Technical Efficacy Thesaurus, Application Domain, Technology Topic.
© 2024 PatSnap. All rights reserved.Legal|Privacy policy|Modern Slavery Act Transparency Statement|Sitemap