A multi-view facial expression recognition method based on mobile terminal
A facial expression recognition and multi-view technology, applied in the field of facial expression recognition, can solve the problem of large deep learning models and achieve the effect of improving recognition accuracy
- Summary
- Abstract
- Description
- Claims
- Application Information
AI Technical Summary
Problems solved by technology
Method used
Image
Examples
Embodiment 1
[0031] The embodiment of the present invention provides an expression attention region learning based on multi-view facial expression recognition on a mobile terminal, including the following steps:
[0032] S1. Cut out part of the face image area from each picture, and perform data enhancement to obtain a data set for training the AA-MDNet model;
[0033] Data enhancement includes random cropping, panning, flipping, color dithering, brightness changes, saturation changes, contrast changes, and sharpness changes.
[0034] S2. Use the GAN model to extend the data set obtained in step S1;
[0035] The GAN model includes four parts: generative model G, image discrimination model D ep , Identity Discrimination Model D id And expression classifier C, generative model G includes encoder G e And decoder G d ; Encoder G e And decoder G d The input data is encoded, analyzed, decoded and reconstructed to generate images, both of which are composed of convolutional layers and fully connected lay...
Embodiment 2
[0042] The embodiment of the present invention provides a posture and expression classification example of a multi-view facial expression recognition method based on a mobile terminal, including:
[0043] 1. Data preprocessing
[0044] Data enhancement: The data sets used to train the AA-MDNet model are KDEF, BU-3DFE and SFEW. In order to better perform expression classification, before starting to train AA-MDNet, it is necessary to perform data enhancement on face images to increase the diversity of samples and minimize interference factors. First, for a picture, crop out part of the face image to reduce other interference factors (background, etc.). During training, perform data enhancement (random cropping, translation, flipping, color jitter, brightness change, saturation change, contrast change, sharpness change) to improve the generalization ability of the model, prevent overfitting, and improve accuracy.
[0045] Generative Adversarial Network (GAN) Extended Data Set: The SF...
Embodiment 3
[0089] The training process of a mobile-based multi-view facial expression recognition method is implemented as follows:
[0090] GAN model training: GAN is used to enrich the data set. Before training AA-MDNet, train the GAN model and save the model file.
[0091] (1) GAN model loss value calculation
[0092] The loss value of the generative model G: Since the generative model is directly related to the two decision models, its own loss value is combined with the encoder G e And decoder G d The loss value of can better train the model, the calculation formula is as follows
[0093] loss G =loss EG +aloss G_ep +bloss E_id
[0094] Among them, the values of a and b are very small, and the default is 0.0001; loss EG , Loss G_ep , Loss E_id Represents the loss value of the generative model, the loss value of the encoder and the loss value of the decoder, respectively.
[0095] Discriminant model D ep The loss value:
[0096] loss D_ep =loss D_ep_input +loss D_ep_G
[0097] Where lo...
PUM
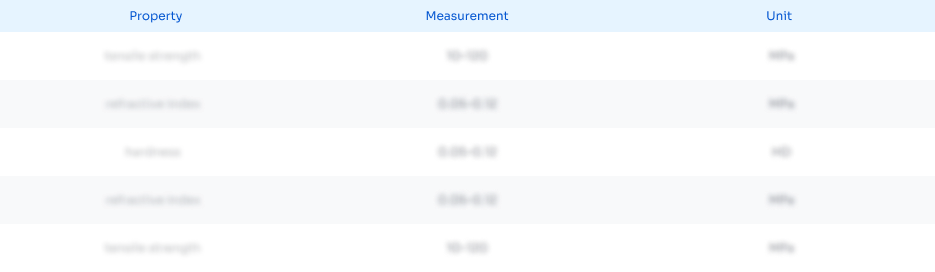
Abstract
Description
Claims
Application Information
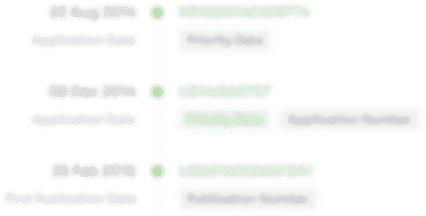
- R&D
- Intellectual Property
- Life Sciences
- Materials
- Tech Scout
- Unparalleled Data Quality
- Higher Quality Content
- 60% Fewer Hallucinations
Browse by: Latest US Patents, China's latest patents, Technical Efficacy Thesaurus, Application Domain, Technology Topic, Popular Technical Reports.
© 2025 PatSnap. All rights reserved.Legal|Privacy policy|Modern Slavery Act Transparency Statement|Sitemap|About US| Contact US: help@patsnap.com