A Real-time Incremental and Adaptive Clustering Method Based on Automotive Radar Data
A technology of automotive radar and data, which is applied in the field of real-time clustering processing of automotive radar data, can solve the problems of low clustering efficiency, unevenness, and inability to cope with data density clusters of automotive radar data, and solve the problem of uneven density of automotive radar data clusters. Uniform, time-saving, and clustering efficiency-enhancing effects
- Summary
- Abstract
- Description
- Claims
- Application Information
AI Technical Summary
Problems solved by technology
Method used
Image
Examples
Embodiment
[0028] Refer to attached figure 1 , a real-time incremental and self-adaptive clustering method based on automotive radar data in the present invention, the innovation point is: improving the DBSCAN algorithm, combining EKF and DBSCAN to realize real-time clustering of automotive radar data.
[0029] attached figure 1 Among them, the improved DBSCAN algorithm of S3, the clustering standard is distance-angle two-dimensional data. In this embodiment, the pseudocode of the improved DBSCAN algorithm is as follows:
[0030] Algorithm 1 Improved Pseudocode of DBSCAN Algorithm
[0031]
[0032] Algorithm 2 Expand_cluster function
[0033]
[0034] Algorithm 1 gives the pseudocode of the improved DBSCAN algorithm, and Algorithm 2 gives the pseudocode of the subfunction Expand_cluster. The input data set is D, the initial distance radius is ε, the point threshold is MinPts, the Kalman prediction set is K, and the real-time data of the automobile 77Ghz millimeter-wave radar ...
PUM
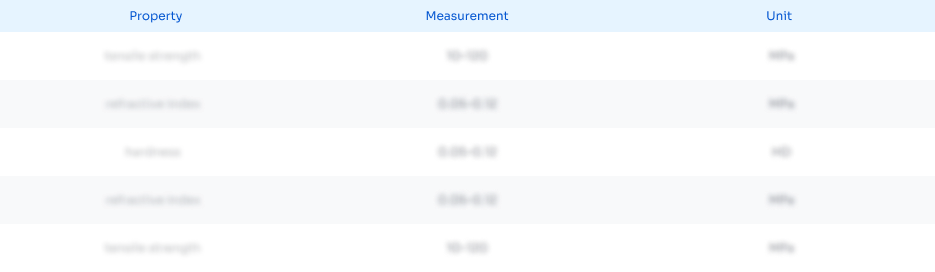
Abstract
Description
Claims
Application Information
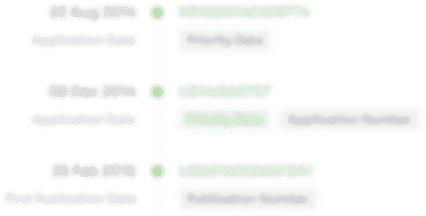
- R&D
- Intellectual Property
- Life Sciences
- Materials
- Tech Scout
- Unparalleled Data Quality
- Higher Quality Content
- 60% Fewer Hallucinations
Browse by: Latest US Patents, China's latest patents, Technical Efficacy Thesaurus, Application Domain, Technology Topic, Popular Technical Reports.
© 2025 PatSnap. All rights reserved.Legal|Privacy policy|Modern Slavery Act Transparency Statement|Sitemap|About US| Contact US: help@patsnap.com