A pavement type estimation method based on depth convolution neural network without loss function
A technology of deep convolution and neural network, applied in the field of road surface type estimation of deep convolutional neural network, can solve the problems of limited estimation conditions, limited estimation conditions, and single recognized road surface type, and achieve simplified feature extraction, The effect of reducing difficulty and improving classification efficiency
- Summary
- Abstract
- Description
- Claims
- Application Information
AI Technical Summary
Problems solved by technology
Method used
Image
Examples
Embodiment Construction
[0076] The present invention will be further described in detail below in conjunction with the accompanying drawings, so that those skilled in the art can implement it with reference to the description.
[0077] Such as figure 1 , 2 As shown, the present invention provides a method for estimating road surface type based on a deep convolutional neural network without loss function, comprising the following steps:
[0078] Step 1: Collect road condition images, calibrate the road surface type and establish a road surface condition database (that is, the road surface type has been determined so as to be used as a training sample for training).
[0079] Step 2: Perform denoising and white balance preprocessing on the input image, reduce the noise in the digital image and correct the image with color cast.
[0080] Step 3: Train the deep convolutional neural network based on the lossless function of the image. First, perform the convolution kernel training of the first layer of p...
PUM
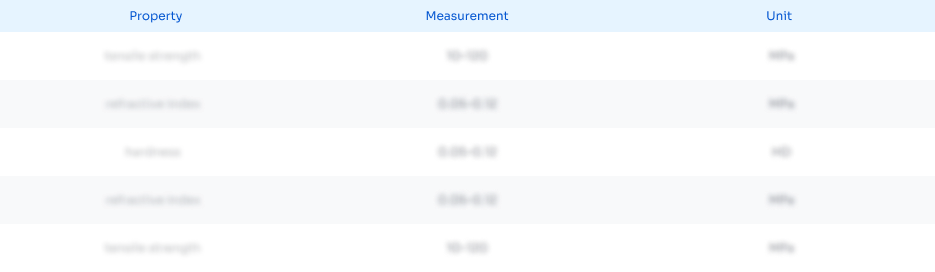
Abstract
Description
Claims
Application Information
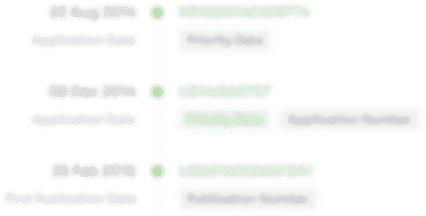
- R&D Engineer
- R&D Manager
- IP Professional
- Industry Leading Data Capabilities
- Powerful AI technology
- Patent DNA Extraction
Browse by: Latest US Patents, China's latest patents, Technical Efficacy Thesaurus, Application Domain, Technology Topic.
© 2024 PatSnap. All rights reserved.Legal|Privacy policy|Modern Slavery Act Transparency Statement|Sitemap