A super-resolution reconstruction method based on feature fusion of dual-channel convolution network
A super-resolution reconstruction and convolutional network technology, applied in the field of super-resolution reconstruction based on dual-channel convolutional network feature fusion, can solve the problem of insufficient extraction of image local features, degradation of image reconstruction quality, and degradation of robustness, etc. problem, achieve the effect of saving preprocessing, simple and convenient reconstruction, and improving robustness
- Summary
- Abstract
- Description
- Claims
- Application Information
AI Technical Summary
Problems solved by technology
Method used
Image
Examples
Embodiment 1
[0038] The embodiment of the present invention proposes a super-resolution reconstruction method based on dual-channel convolutional network feature fusion, see figure 1 and figure 2 , the method includes the following steps:
[0039] 101: Build a dual-channel convolutional network based on a dense convolutional network with different convolution kernels;
[0040] Among them, the two-channel convolutional network includes: two sub-channels, each sub-channel adopts a densely connected network structure, which is generated by cascading multiple densely connected blocks, and each densely connected block is connected by a 1×1 convolutional layer. It consists of a 3×3 convolutional layer and a skip layer connection, and uses a PReLU layer as a nonlinear activation function before each convolutional layer.
[0041] 102: Use weighted L 1 The norm is used as a loss function, and the image is super-resolution reconstructed after each sub-channel, and the loss function is calculated...
Embodiment 2
[0055] The scheme in embodiment 1 is further introduced below in conjunction with specific mathematical formulas and examples, see the following description for details:
[0056] 201: constructing a data set;
[0057] Wherein, the step 201 includes:
[0058] Step 1: Divide the data set. The source of the data set used is DIV2K (DIVerse 2K resolution images, a variety of 2000 resolution images). Each sample includes: high resolution and low resolution images of different scales (as training images) , the low-resolution image is generated by a degradation method. The DIV2K data set divided by the embodiment of the present invention includes 800 pieces of training data and 100 pieces of verification data.
[0059] Wherein, the degrading method may be: commonly used algorithms such as bicubic difference downsampling and bilinear downsampling.
[0060] The second step: image cropping, the training image is cropped into several 96×96 image blocks, which are used as the input of t...
Embodiment 3
[0085] Below in conjunction with concrete experimental data, the scheme in embodiment 1 and 2 is further introduced, see the following description for details:
[0086] 301: Data preparation:
[0087] Among them, this step includes:
[0088] (a) Divide the dataset:
[0089] This embodiment uses the DIV2K data set, including: 800 training images, 100 verification images, and 100 test images. Since the test set does not disclose labels, this paper uses the validation set as the test evaluation data.
[0090] (b) Randomly crop 800 training images into 96×96 image blocks, which are used as network input in the training phase.
[0091] 302: Network structure construction;
[0092] The network structure in the embodiment of the present invention can be divided into: a feature pre-extraction module, two sub-channels (containing several dense convolution blocks respectively), a feature fusion module, a layer-skip connection, and a feature reconstruction module (including feature u...
PUM
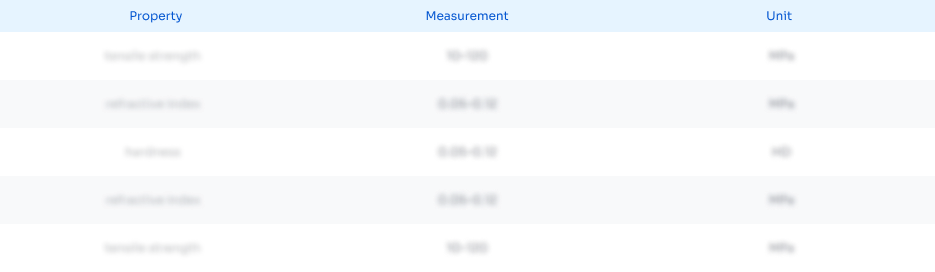
Abstract
Description
Claims
Application Information
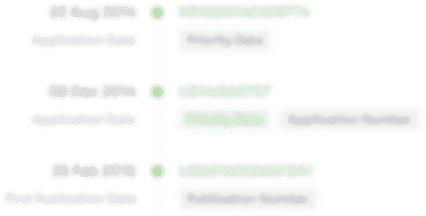
- R&D Engineer
- R&D Manager
- IP Professional
- Industry Leading Data Capabilities
- Powerful AI technology
- Patent DNA Extraction
Browse by: Latest US Patents, China's latest patents, Technical Efficacy Thesaurus, Application Domain, Technology Topic, Popular Technical Reports.
© 2024 PatSnap. All rights reserved.Legal|Privacy policy|Modern Slavery Act Transparency Statement|Sitemap|About US| Contact US: help@patsnap.com