A three-dimensional pulmonary nodule recognition method based on convolution neural network
A technology of convolutional neural network and identification method, which is applied in the field of three-dimensional pulmonary nodule identification based on convolutional neural network, can solve problems such as gradient disappearance, degradation, and gradient explosion, and achieve the effect of improving recognition accuracy and increasing accuracy
- Summary
- Abstract
- Description
- Claims
- Application Information
AI Technical Summary
Problems solved by technology
Method used
Image
Examples
Embodiment Construction
[0020] The specific embodiments of the present invention will be described in further detail below in conjunction with the accompanying drawings, but the embodiments of the present invention are not limited thereto.
[0021] The basic principle of the technology of the present invention is: Squeeze-and-Excitation Net (SENet) contains a structural unit - Squeeze-and-Excitation (SE) unit (also known as Squeeze excitation block, SE block, SEblock), which can greatly improve neural network performance.
[0022] The DenseNet network structure is mainly composed of dense blocks. The bottleneck layer (bottleneck layer) and transition layer can be added to DenseNet to reduce dimensionality and reduce the amount of calculation. Each layer of DenseNet takes the output of the previous layer as input. For a traditional network with L layers, there are a total of L connections, and for DenseNet there are (L×(L+1)) / 2 connections. The input of each layer in the dense block comes from the ou...
PUM
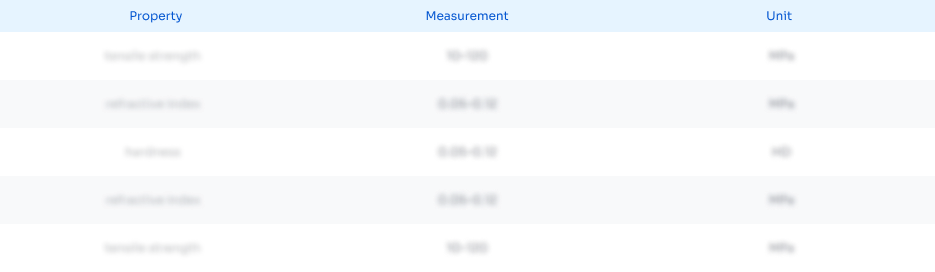
Abstract
Description
Claims
Application Information
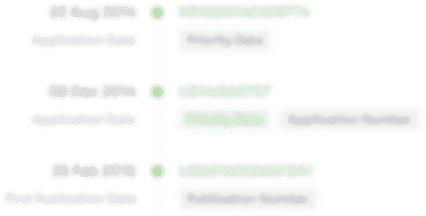
- R&D Engineer
- R&D Manager
- IP Professional
- Industry Leading Data Capabilities
- Powerful AI technology
- Patent DNA Extraction
Browse by: Latest US Patents, China's latest patents, Technical Efficacy Thesaurus, Application Domain, Technology Topic, Popular Technical Reports.
© 2024 PatSnap. All rights reserved.Legal|Privacy policy|Modern Slavery Act Transparency Statement|Sitemap|About US| Contact US: help@patsnap.com