Text multi-label classification method based on semantic unit information
A technology of semantic units and classification methods, applied in text database clustering/classification, semantic tool creation, unstructured text data retrieval, etc., can solve problems such as insufficient attention mechanism noise affecting classification contribution, etc., and achieve parameter increment Small, less parameter cost and time cost, and the effect of improving accuracy
- Summary
- Abstract
- Description
- Claims
- Application Information
AI Technical Summary
Problems solved by technology
Method used
Image
Examples
Embodiment Construction
[0038] Below in conjunction with accompanying drawing, further describe the present invention through embodiment, but do not limit the scope of the present invention in any way.
[0039] The present invention provides a text multi-label classification method based on semantic unit information, which improves the sequence-to-sequence model based on the attention mechanism as the baseline model, improves the content that the attention mechanism focuses on, and improves the attention mechanism at the source Represents and improves the contribution of attention-based sequence-to-sequence models in text multi-label classification.
[0040] The baseline model adopted by the model SU4MLC proposed by the present invention is the sequence-to-sequence model of the cyclic neural network based on the attention mechanism, and the cyclic neural network adopts the LSTM. figure 1 It is a schematic flow chart of the method of the present invention. The specific implementation steps are as fol...
PUM
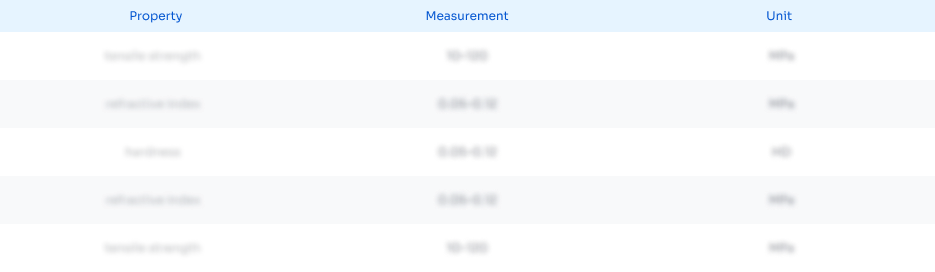
Abstract
Description
Claims
Application Information
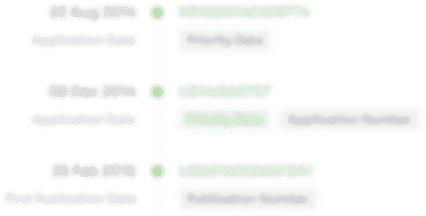
- R&D Engineer
- R&D Manager
- IP Professional
- Industry Leading Data Capabilities
- Powerful AI technology
- Patent DNA Extraction
Browse by: Latest US Patents, China's latest patents, Technical Efficacy Thesaurus, Application Domain, Technology Topic, Popular Technical Reports.
© 2024 PatSnap. All rights reserved.Legal|Privacy policy|Modern Slavery Act Transparency Statement|Sitemap|About US| Contact US: help@patsnap.com