An aluminum electrolysis superheat degree identification method based on a semi-supervised extreme learning machine
A technology of extreme learning machine and recognition method, which is applied in the field of aluminum electrolytic superheat recognition based on semi-supervised extreme learning machine, can solve the problems of fast training speed and weak inference power, and achieve the effect of fast training speed and overcoming weak inference power
- Summary
- Abstract
- Description
- Claims
- Application Information
AI Technical Summary
Problems solved by technology
Method used
Image
Examples
Embodiment 1
[0064] Such as figure 1 As shown, the present invention provides a kind of aluminum electrolysis superheat identification method based on semi-supervised extreme learning machine, and described method comprises:
[0065] S1. Collect real-time production data of aluminum electrolysis, and normalize and standardize the collected data;
[0066] S2. Construct a Hessian regularization operator;
[0067] S3, calculating the hidden layer output of the extreme learning machine ELM model;
[0068] S4. Construct the loss function of the ELM model, and solve the output weight matrix.
[0069] The method of the present invention is described in further detail below.
[0070] 1. Extreme Learning Machine
[0071] Extreme learning machine is a training algorithm to effectively determine the parameters of single hidden layer feedforward neural network. When performing hierarchical extreme learning machine training, it is assumed that there are input variables X∈R N×m and the output vari...
Embodiment 2
[0120] Efficient aluminum electrolysis process has always been a challenging industrial problem. However, due to various external conditions and complex physical and chemical reactions and interferences of manual operations, the aluminum electrolysis process often works in many different working conditions, which increases the difficulty of monitoring the aluminum electrolysis process. In the process of aluminum electrolysis, the degree of superheat is mainly identified by manual observation of the electrolyte, fire eyes, etc. The online identification method of the superheat of the electrolytic cell has not yet been solved. Therefore, 12 process variables are selected for soft sensor modeling. The data set comes from the real-time production data of two 400KA electrolytic cells of Shandong Weiqiao Aluminum Co., Ltd., and 1200 daily data samples of each of the two electrolytic cells are selected as training data, of which 500 are labeled data for training models, and the other ...
PUM
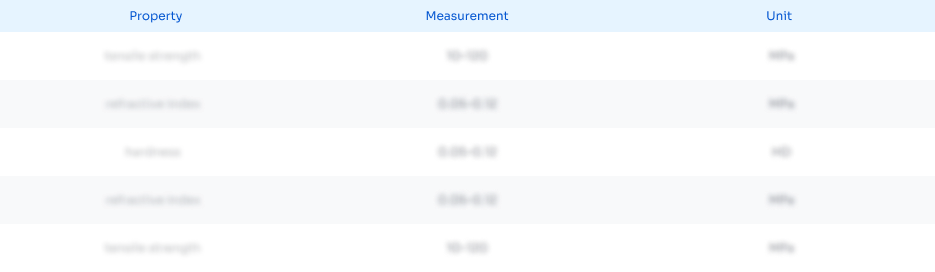
Abstract
Description
Claims
Application Information
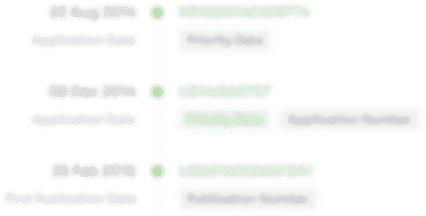
- R&D
- Intellectual Property
- Life Sciences
- Materials
- Tech Scout
- Unparalleled Data Quality
- Higher Quality Content
- 60% Fewer Hallucinations
Browse by: Latest US Patents, China's latest patents, Technical Efficacy Thesaurus, Application Domain, Technology Topic, Popular Technical Reports.
© 2025 PatSnap. All rights reserved.Legal|Privacy policy|Modern Slavery Act Transparency Statement|Sitemap|About US| Contact US: help@patsnap.com