Traffic flow prediction method based on data-driven k-nearest non-parametric regression
A non-parametric regression and data-driven technology, which is applied in the field of intelligent transportation system and Internet of Vehicles, can solve the problems of low efficiency of KNN prediction method and long execution time of KNN, so as to reduce the time of searching historical data, ensure the accuracy rate, and reduce the execution time. the effect of time
- Summary
- Abstract
- Description
- Claims
- Application Information
AI Technical Summary
Problems solved by technology
Method used
Image
Examples
Embodiment Construction
[0031] Below in conjunction with accompanying drawing and specific embodiment, further illustrate the present invention, should be understood that these examples are only for illustrating the present invention and are not intended to limit the scope of the present invention, after having read the present invention, those skilled in the art will understand various aspects of the present invention All modifications of the valence form fall within the scope defined by the appended claims of the present application.
[0032] A data-driven k-neighborhood non-parametric regression based traffic flow forecasting method, developed based on a two-step data search algorithm, first seeks and identifies candidate input data from historical databases to approximate the current state during the non-prediction period, and then The best decision input data for prediction is identified from the candidate input data at the prediction point, and finally the best decision input data is used to gen...
PUM
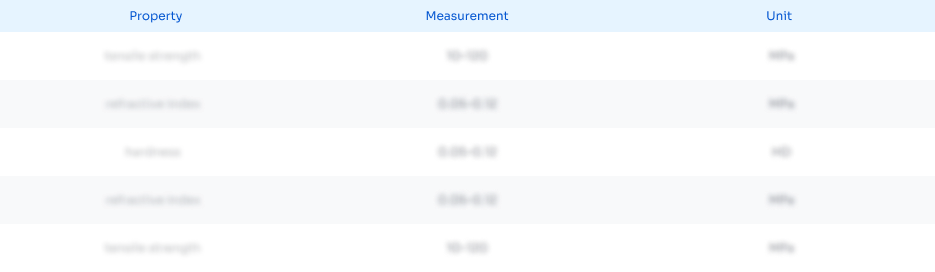
Abstract
Description
Claims
Application Information
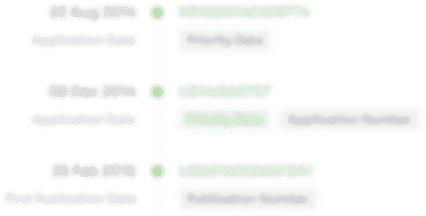
- R&D Engineer
- R&D Manager
- IP Professional
- Industry Leading Data Capabilities
- Powerful AI technology
- Patent DNA Extraction
Browse by: Latest US Patents, China's latest patents, Technical Efficacy Thesaurus, Application Domain, Technology Topic, Popular Technical Reports.
© 2024 PatSnap. All rights reserved.Legal|Privacy policy|Modern Slavery Act Transparency Statement|Sitemap|About US| Contact US: help@patsnap.com