Composite fault diagnosis method and device based on a multi-label classification convolutional neural network
A technology of convolutional neural network and composite faults, which is applied in neural learning methods, biological neural network models, neural architectures, etc., can solve problems such as increased model complexity, insufficient data volume of composite faults, and increased number of model parameters, achieving excellent results. Similarity of the same kind and difference of different kinds, good decoupling, and the effect of good feature learning ability
- Summary
- Abstract
- Description
- Claims
- Application Information
AI Technical Summary
Problems solved by technology
Method used
Image
Examples
Embodiment
[0049] A one-dimensional convolutional neural network compound fault diagnosis method based on multi-label classifier, the algorithm flow is as follows figure 1As shown, the method uses the Margin Loss function to expand the distance between classes and reduce the distance between classes, and improves the cost function and activation function of the one-dimensional convolutional neural network. Output multiple labels. The method includes steps as follows:
[0050] Step 1: Collect vibration acceleration signals of rotating machinery under single fault and composite fault conditions, and set a certain sample length and sample overlap rate to truncate and extract a large number of samples;
[0051] Step 2: For each sample, a single label is given for a single fault, and multiple labels are given for a compound fault, and then the sample set is randomly divided into a training set and a test set according to a certain proportion;
[0052] Step 3: Use Keras to build a deep one-d...
PUM
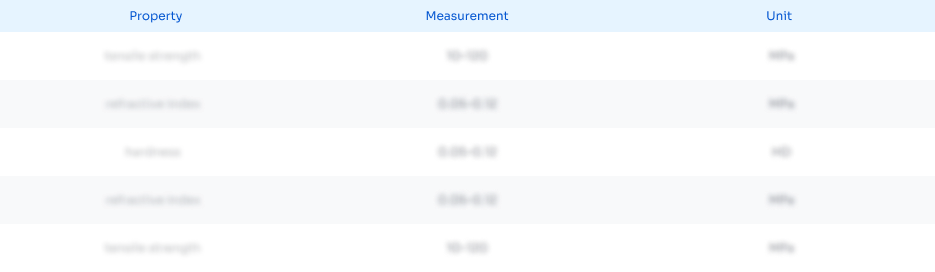
Abstract
Description
Claims
Application Information
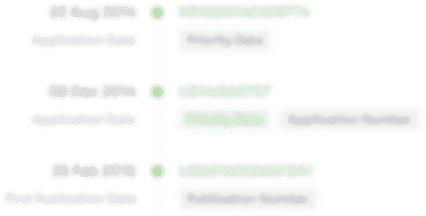
- R&D Engineer
- R&D Manager
- IP Professional
- Industry Leading Data Capabilities
- Powerful AI technology
- Patent DNA Extraction
Browse by: Latest US Patents, China's latest patents, Technical Efficacy Thesaurus, Application Domain, Technology Topic.
© 2024 PatSnap. All rights reserved.Legal|Privacy policy|Modern Slavery Act Transparency Statement|Sitemap