An Image Super-Resolution Reconstruction Method Based on Wavelet Coefficient Learning
A super-resolution reconstruction and wavelet coefficient technology, applied in the field of image super-resolution based on wavelet coefficient learning, can solve the problems of long training period, large amount of calculation, lack of detailed information, etc., to reduce calculation burden, increase sparsity, The effect of enhancing the effect
- Summary
- Abstract
- Description
- Claims
- Application Information
AI Technical Summary
Problems solved by technology
Method used
Image
Examples
Embodiment 1
[0024] Embodiment 1: several different pictures are processed according to the above method, and the specific steps are as follows:
[0025] (1) Select a training set commonly used in super-resolution methods. The training set in this embodiment uses 800 2k resolution images in DIV2K and 2650 2k resolution images in Flickr2K. Perform bicubic interpolation and downsampling to obtain the corresponding low-resolution image. When the magnification is 4, the image is cut into 48×48 low-resolution image blocks Y LR , corresponding to intercepting a high-resolution image block Y of size 192×192 HR , and randomly intercept image blocks of a certain size to generate high-resolution image blocks Y HRand its corresponding low-resolution image patch Y LR , and randomly flip and rotate it by 90 degrees, which keeps the total number of images the same while adding different image forms. In order to reduce the amount of calculation, only the Y channel in the Ycbcr format is used for the c...
PUM
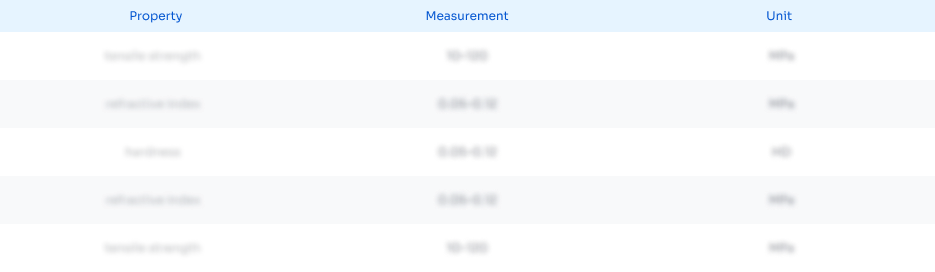
Abstract
Description
Claims
Application Information
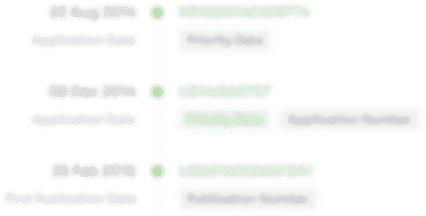
- R&D Engineer
- R&D Manager
- IP Professional
- Industry Leading Data Capabilities
- Powerful AI technology
- Patent DNA Extraction
Browse by: Latest US Patents, China's latest patents, Technical Efficacy Thesaurus, Application Domain, Technology Topic.
© 2024 PatSnap. All rights reserved.Legal|Privacy policy|Modern Slavery Act Transparency Statement|Sitemap