Adaptive graph regularization non-negative matrix factorization method for face recognition
A non-negative matrix decomposition, face recognition technology, applied in the field of adaptive graph regularization non-negative matrix decomposition, can solve the problems of low accuracy of face recognition, damage to local neighborhood structure information, etc., to remove noise and outliers Value, local neighborhood structure information is complete, and the effect of improving the recognition accuracy
- Summary
- Abstract
- Description
- Claims
- Application Information
AI Technical Summary
Problems solved by technology
Method used
Image
Examples
Embodiment Construction
[0024] The present invention will be further described below in conjunction with the accompanying drawings.
[0025] The processing procedure of the non-negative matrix factorization (NMF) described in the present invention is: face photo all carries out matrix processing, obtains data matrix X, X=[X 1 ,X 2 ,...,X n ]∈R m×n , where X i (i=1,2,...n) represents i data points, each data point is an m-dimensional vector, and there are n data samples in total.
[0026] For NMF, the aim is to find the product of two non-negative matrices to represent the original data matrix.
[0027] X≈UV T (1)
[0028] where U ∈ R m×k is the basis matrix, V∈R n×k is the coefficient matrix. When clustering, set k as the number of clusters, and each column of matrix U is a basis vector. Based on the basis matrix U, any data point is reconstructed from the data X by using different linear combinations of these k-column vectors in V. Each row vector of matrix V is the weight coefficient of...
PUM
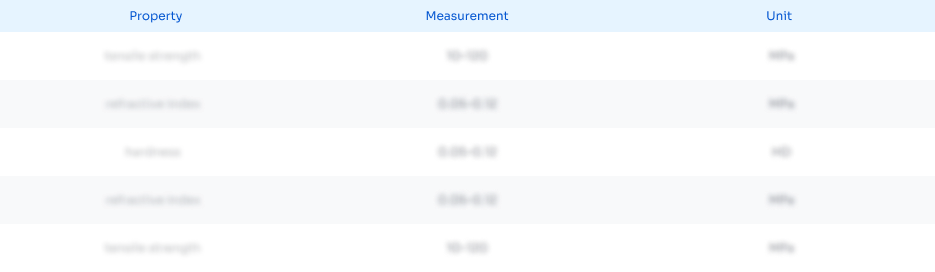
Abstract
Description
Claims
Application Information
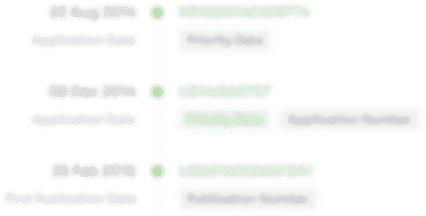
- R&D Engineer
- R&D Manager
- IP Professional
- Industry Leading Data Capabilities
- Powerful AI technology
- Patent DNA Extraction
Browse by: Latest US Patents, China's latest patents, Technical Efficacy Thesaurus, Application Domain, Technology Topic, Popular Technical Reports.
© 2024 PatSnap. All rights reserved.Legal|Privacy policy|Modern Slavery Act Transparency Statement|Sitemap|About US| Contact US: help@patsnap.com