A cross-domain federated learning model and method based on a value iteration network
A learning model and cross-domain technology, applied in the field of cross-domain federated learning models based on value iterative networks, it can solve the problems of strong generalization ability, no reduction in the amount of parameters, and low domain similarity, so as to protect data privacy and overcome computing problems. Complexity and cost consumption, the effect of improving forecast accuracy
- Summary
- Abstract
- Description
- Claims
- Application Information
AI Technical Summary
Problems solved by technology
Method used
Image
Examples
Embodiment Construction
[0037] The following describes the implementation of the present invention through specific specific examples in conjunction with the accompanying drawings. Those skilled in the art can easily understand other advantages and effects of the present invention from the content disclosed in this specification. The present invention can also be implemented or applied through other different specific examples, and various details in this specification can also be based on different viewpoints and applications, and various modifications and changes can be made without departing from the spirit of the present invention.
[0038] Before introducing the present invention, the abbreviations and key terms involved in the present invention are defined as follows:
[0039] Deep learning: Deep learning was proposed by Hinton et al. in 2006 and is a new field of machine learning. Deep learning is introduced into machine learning to bring it closer to the original goal-artificial intelligence. Dee...
PUM
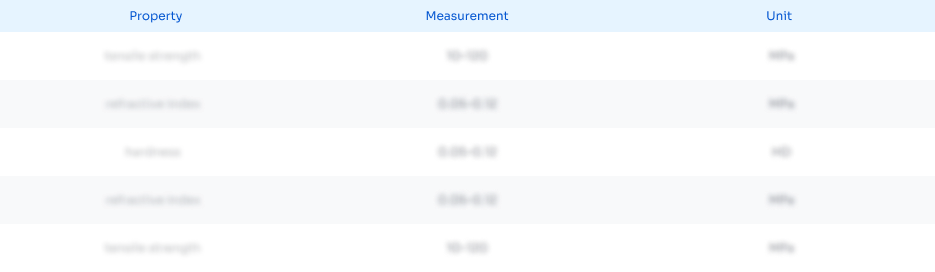
Abstract
Description
Claims
Application Information
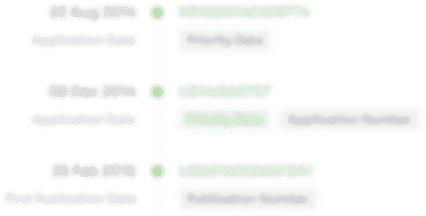
- R&D Engineer
- R&D Manager
- IP Professional
- Industry Leading Data Capabilities
- Powerful AI technology
- Patent DNA Extraction
Browse by: Latest US Patents, China's latest patents, Technical Efficacy Thesaurus, Application Domain, Technology Topic, Popular Technical Reports.
© 2024 PatSnap. All rights reserved.Legal|Privacy policy|Modern Slavery Act Transparency Statement|Sitemap|About US| Contact US: help@patsnap.com