A CNN and LSTM-based rolling bearing residual service life prediction method
A rolling bearing and life prediction technology, applied in mechanical bearing testing, character and pattern recognition, special data processing applications, etc., can solve gradual failures and sudden failures without considering the performance degradation of rolling bearings, affecting prediction accuracy, and health indicators cannot be taken into account at the same time and other problems, to achieve good monotonic trend and eliminate the effect of local oscillation
- Summary
- Abstract
- Description
- Claims
- Application Information
AI Technical Summary
Problems solved by technology
Method used
Image
Examples
Embodiment Construction
[0046] combine Figure 1 to Figure 13 In this embodiment, the implementation and effect verification of a rolling bearing RUL prediction method based on a convolutional neural network (CNN) and a long-short-term memory neural network (LSTM) proposed by the present invention are described as follows:
[0047] 1 Related Deep Learning Theory
[0048] 1.1 Convolutional Neural Network
[0049] CNN is composed of multiple convolutional layers and multiple pooling layers stacked. A single-layer CNN network consists of two layers: a convolutional layer and a pooling layer, which directly process raw input sequences. Such as figure 1 As shown, each layer of CNN contains several convolution kernels of the same size and the same type of pooling function. First, the convolution kernel traverses the entire input sequence data to generate a higher-level, more abstract feature space. Then, the pooling layer compresses each generated feature for secondary feature extraction, dimensionali...
PUM
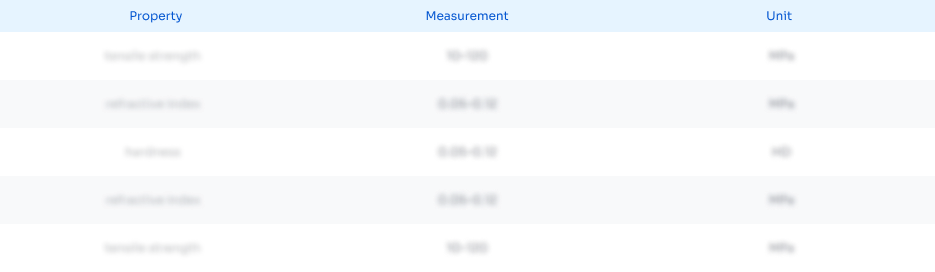
Abstract
Description
Claims
Application Information
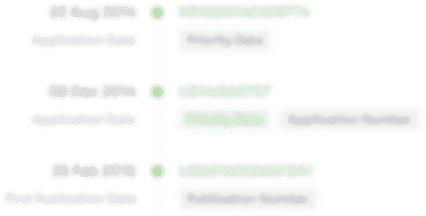
- R&D Engineer
- R&D Manager
- IP Professional
- Industry Leading Data Capabilities
- Powerful AI technology
- Patent DNA Extraction
Browse by: Latest US Patents, China's latest patents, Technical Efficacy Thesaurus, Application Domain, Technology Topic, Popular Technical Reports.
© 2024 PatSnap. All rights reserved.Legal|Privacy policy|Modern Slavery Act Transparency Statement|Sitemap|About US| Contact US: help@patsnap.com