A collaborative tumor segmentation method for t1wi-fMRI images based on 3D-unet and graph theory segmentation
A collaborative segmentation and tumor technology, applied in the field of image processing, can solve the problems that it is difficult to express all the information of the tumor, and cannot accurately describe the tumor boundary, so as to achieve the effect of accurate description
- Summary
- Abstract
- Description
- Claims
- Application Information
AI Technical Summary
Problems solved by technology
Method used
Image
Examples
Embodiment Construction
[0027] In order to make the object, technical solution and advantages of the present invention clearer, the present invention will be further explained below in conjunction with specific implementation and accompanying drawings.
[0028] refer to figure 1 and figure 2 , a T1WI-fMRI image tumor collaborative segmentation method based on 3D-Unet and graphic segmentation, which can make full use of the information between multi-modal MRIs to realize automatic and accurate description of tumors and tumor subregions, including the following steps:
[0029] Step 1. Image preprocessing: Obtain the MRI training data set, interpolate the MRI images of different modalities to the same voxel size through the bilinear interpolation algorithm, and use the registration algorithm based on B-splines to align the T1WI and the corresponding fMRI space Align the coordinates, and further generate the corresponding brain tissue / non-brain area mask through gray value thresholding;
[0030] Step ...
PUM
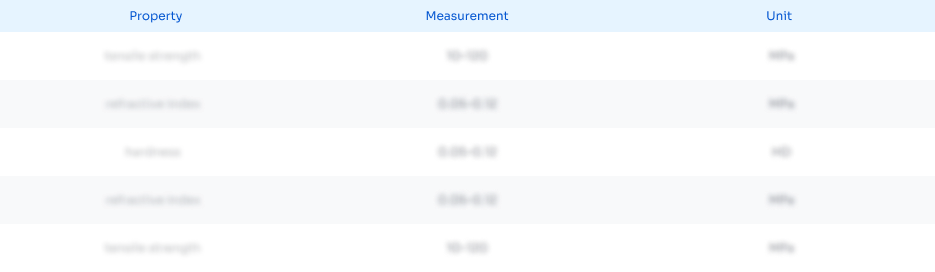
Abstract
Description
Claims
Application Information
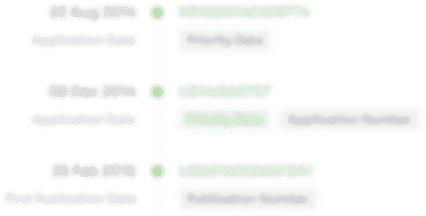
- R&D Engineer
- R&D Manager
- IP Professional
- Industry Leading Data Capabilities
- Powerful AI technology
- Patent DNA Extraction
Browse by: Latest US Patents, China's latest patents, Technical Efficacy Thesaurus, Application Domain, Technology Topic, Popular Technical Reports.
© 2024 PatSnap. All rights reserved.Legal|Privacy policy|Modern Slavery Act Transparency Statement|Sitemap|About US| Contact US: help@patsnap.com