A fast image super-resolution reconstruction method based on deep learning
A technology of super-resolution reconstruction and deep learning, which is applied to the improved algorithm of the training process of the deep network dual-branch structure, and the field of fast image super-resolution reconstruction, which can solve the problem of large memory space load, reduce the ringing phenomenon, reduce training Effects of reduced burden and good edge information
- Summary
- Abstract
- Description
- Claims
- Application Information
AI Technical Summary
Problems solved by technology
Method used
Image
Examples
Embodiment 1
[0035] Embodiment 1: as Figure 1-5 As shown, a fast image super-resolution reconstruction method based on deep learning includes the following steps:
[0036] Step1, select the image training set and test set, and process to obtain low-resolution image blocks and high-resolution image blocks; in the step Step1, select the high-resolution image BSD200 data set that includes 200 png format and 91 bmp format for use The image set Train91 is used as the model training set, and Set5, Set14, BSD100, and Urban100 are selected as the standard test set; in order to solve the problem of excessive memory space load, when the magnification factor is 3, the image is cut into 48×48 low-resolution images Image block (Low Resolution, LR) and the corresponding 144×144 high-resolution image block y HR (High Resolution, HR);
[0037] Step2. Use the deep neural network to perform feature extraction on the low-resolution image, refine the feature of the nested network, and sub-pixel up-sampling...
Embodiment 2
[0049] Embodiment 2: as Figure 1-5 As shown, a fast image super-resolution reconstruction method based on deep learning includes the following steps:
[0050] Step1, select the training set and test set according to the model training scheme and process it: the present invention continues to use the commonly used training data sets for image super-resolution reconstruction, i.e. BSD200 and Train91, wherein the former contains 200 high-resolution natural images in png format The scene image, which contains 91 high-resolution flower images in bmp format.
[0051] In the training data preparation stage, considering that the image set is too small (a total of 291 images) is prone to overfitting, the training data set is enhanced to generate a data set eight times larger than the original image (a total of 2328 images); In order to solve the problem of excessive memory space load, when the magnification factor is 3, the training image is cut into low-resolution image blocks of 48...
PUM
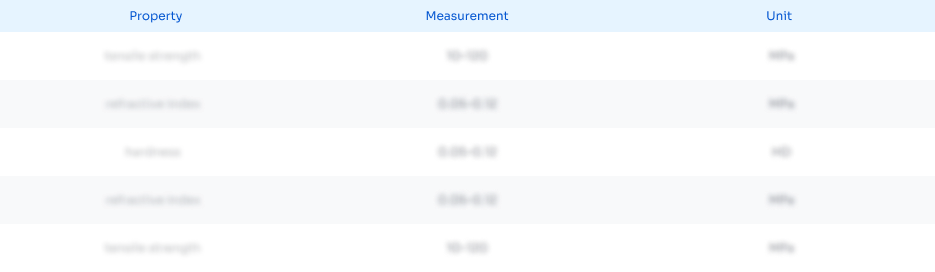
Abstract
Description
Claims
Application Information
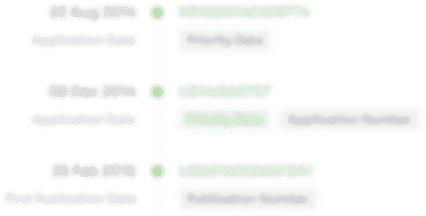
- R&D
- Intellectual Property
- Life Sciences
- Materials
- Tech Scout
- Unparalleled Data Quality
- Higher Quality Content
- 60% Fewer Hallucinations
Browse by: Latest US Patents, China's latest patents, Technical Efficacy Thesaurus, Application Domain, Technology Topic, Popular Technical Reports.
© 2025 PatSnap. All rights reserved.Legal|Privacy policy|Modern Slavery Act Transparency Statement|Sitemap|About US| Contact US: help@patsnap.com