Electric power system state estimation method based on convolutional neural network
A convolutional neural network and state estimation technology, which is applied in neural learning methods, biological neural network models, neural architectures, etc., can solve problems such as high hardware requirements, long computing time, and high computer hardware requirements, and achieve accelerated error reversal The effects of propagation efficiency, speeding up state estimation, and reducing training difficulty
- Summary
- Abstract
- Description
- Claims
- Application Information
AI Technical Summary
Problems solved by technology
Method used
Image
Examples
Embodiment Construction
[0046] The present invention will be described in detail below in conjunction with the accompanying drawings and specific embodiments of the present invention. This embodiment is implemented on the premise of the technical solution of the present invention, and detailed implementation and specific operations are given, but the protection scope of the present invention is not limited to the following embodiments.
[0047] Such as figure 1 As shown, a power system state estimation method based on convolutional neural network is based on a convolutional neural network model. The convolutional neural network model includes the number of layers of the convolutional neural network, the size of the convolution kernel, and the pooling method , activation function, and the number of neurons in each layer of the network, the model of the convolutional neural network specifically includes:
[0048] 1) Design a five-layer convolutional neural network, the network structure is:
[0049] ...
PUM
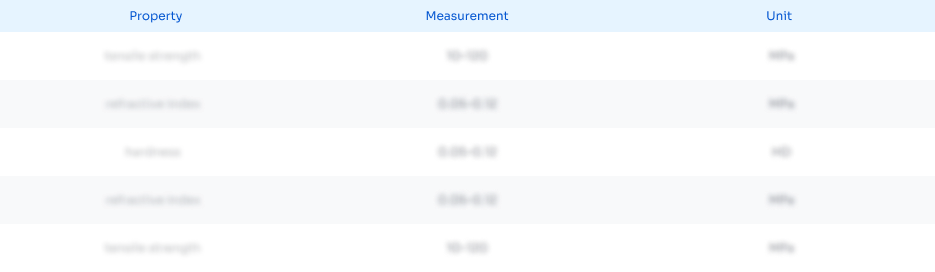
Abstract
Description
Claims
Application Information
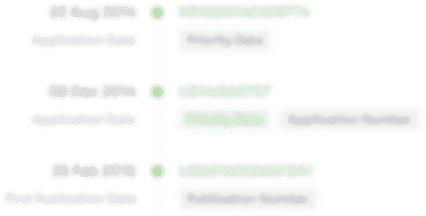
- R&D
- Intellectual Property
- Life Sciences
- Materials
- Tech Scout
- Unparalleled Data Quality
- Higher Quality Content
- 60% Fewer Hallucinations
Browse by: Latest US Patents, China's latest patents, Technical Efficacy Thesaurus, Application Domain, Technology Topic, Popular Technical Reports.
© 2025 PatSnap. All rights reserved.Legal|Privacy policy|Modern Slavery Act Transparency Statement|Sitemap|About US| Contact US: help@patsnap.com