6D pose estimation method based on an instance segmentation network and iterative optimization
A pose estimation and iterative optimization technology, applied in biological neural network models, computing, image analysis, etc., can solve the problems of consuming large computing resources, less texture features, and low time efficiency, and achieve strong adaptability and robustness , Improve detection performance, improve the effect of running speed
- Summary
- Abstract
- Description
- Claims
- Application Information
AI Technical Summary
Problems solved by technology
Method used
Image
Examples
Embodiment
[0117] In order to verify the effect of this scheme, the present invention carried out object recognition experiments and pose estimation experiments respectively, which were used to evaluate the recognition effect of the instance segmentation network and the accuracy of the final output pose.
[0118] In order to verify the effect of object recognition, we conducted experiments on an existing "Shelf&Tote" Benchmark dataset and our own collected dataset. The objects in the "Shelf&Tote" Benchmark dataset are rich in texture features, and we The objects in the data set collected by myself lack texture information, and there are a large number of similar objects stacked and mixed.
[0119] Whether it is the "Shelf&Tote" Benchmark dataset or the self-collected dataset, there are good recognition results.
[0120] In order to evaluate the performance of this method, the pose error is defined as follows:
[0121]
[0122] Based on the attitude error, we define the accuracy rate ...
PUM
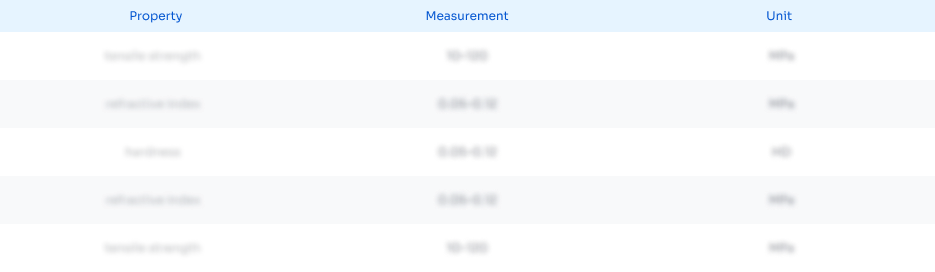
Abstract
Description
Claims
Application Information
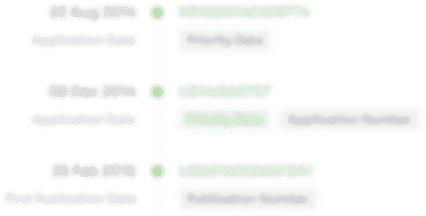
- R&D Engineer
- R&D Manager
- IP Professional
- Industry Leading Data Capabilities
- Powerful AI technology
- Patent DNA Extraction
Browse by: Latest US Patents, China's latest patents, Technical Efficacy Thesaurus, Application Domain, Technology Topic, Popular Technical Reports.
© 2024 PatSnap. All rights reserved.Legal|Privacy policy|Modern Slavery Act Transparency Statement|Sitemap|About US| Contact US: help@patsnap.com