A self-adaptive weight deep learning target classification method based on feature fusion
A technology of self-adaptive weight and feature fusion, applied in the field of image recognition, can solve the problems of not considering the gain of classification accuracy, unsatisfactory classification effect, and different problems, so as to improve the recall rate, excellent detection ability, and high accuracy rate. Effect
- Summary
- Abstract
- Description
- Claims
- Application Information
AI Technical Summary
Problems solved by technology
Method used
Image
Examples
Embodiment Construction
[0041] The following examples describe the present invention in more detail.
[0042] Structural block diagram of the present invention is as figure 1 As shown, it involves Faster Rcnn network, Resnet network, SENet network, where Faster Rcnn network is used to complete the work of target recognition, Resnet network is used to extract image convolution features and HOG features, and SENet network is used to calculate the weight of feature maps Vector, and achieve the target classification task through feature fusion.
[0043] 1. Develop a low threshold-coarse detection strategy
[0044] The present invention uses the Faster-Rcnn target detection network containing the Roi-Align layer and the FPN structure to reduce the detectable threshold of the probability value calculated by the softmax function of the network output node and display more low-probability targets. These targets are used as backup Choose a target. In order to achieve the goal of improving the detection rec...
PUM
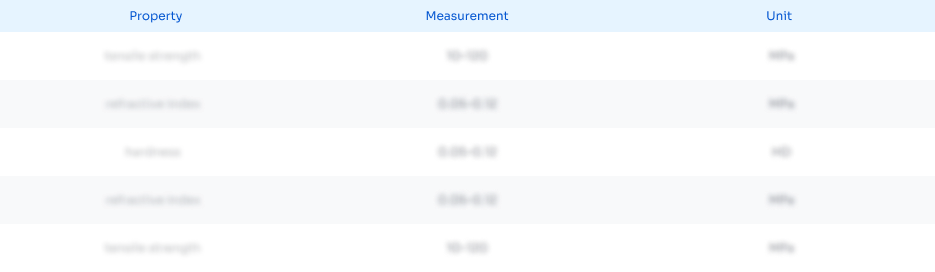
Abstract
Description
Claims
Application Information
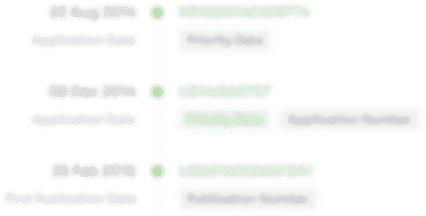
- R&D Engineer
- R&D Manager
- IP Professional
- Industry Leading Data Capabilities
- Powerful AI technology
- Patent DNA Extraction
Browse by: Latest US Patents, China's latest patents, Technical Efficacy Thesaurus, Application Domain, Technology Topic, Popular Technical Reports.
© 2024 PatSnap. All rights reserved.Legal|Privacy policy|Modern Slavery Act Transparency Statement|Sitemap|About US| Contact US: help@patsnap.com